1 Decolonizing Data
The Movement for Indigenous Data Sovereignty
Autumn Asher BlackDeer, PhD, MSW
Abstract
Research has long been used to both harm and further other Indigenous communities. More recent developments have used data, or the perceived absence thereof, as a means to perpetuate Indigenous erasure. American Indian and Alaska Native communities are often relegated to the “Other” racial category in quantitative data, often lumped together with several other racial and ethnic groups, making our communities impossible to see within the data. The National Congress on American Indians describes this as the “Asterisk Nation,” whereas Indigenous communities are typically represented by an asterisk instead of a data point when reporting racial and ethnic data. When Natives are represented in data, there is an issue of ethnic gloss, flattening the vast differences and cultural depths into one conglomerate pan-Indigenous group. Conversely, other issues with Native data include the representation paradox in balancing tribe specificity vs. nationwide generalizability. In order to challenge the dominant deficit-based narratives on Indigenous communities, representative, culturally specific data is needed. Ultimately, there is a growing need to decolonize data. Indigenous data sovereignty is emerging as an ideal solution to address representation gaps and promote tribal sovereignty in research and education practices. This chapter will detail how Indigenous data sovereignty is a vital framework for critical research and teaching practices and delineate steps to decolonize data.
The emergence of the Big Data movement has been described as an unprecedented opportunity to reach populations and communities that have historically been considered too difficult to reach (Anderson et al., 2022). American Indian and Alaska Native communities are often discarded or lost within the Big Data movement through data colonialism, relegated to the “Other” racial category throughout the cycle of erasure, or lost altogether within the representation paradox. This chapter will introduce Indigenous data sovereignty as a pathway to remedy these harms and decolonize data in research and teaching.
Big Data and Data Colonialism
As part of the digital revolution, the Big Data movement has drastically increased the quantity of data (Coulton et al., 2015). There are three “V’s” that contribute to understanding the movement for big data. The first V is Volume – attributed to the increasing amount of data produced daily (Thatcher et al., 2016). The second V is Velocity – which is described as the high speed of data flowing in and out of systems (Thatcher et al., 2016). The third and final V is Variety – which details the ever-broadening range of types of data and data sources (Thatcher et al., 2016). Ultimately, big data entails more data, moving at higher speeds, and multiple types of data and data sources being available at unprecedented rates. Big data has generated wide appeal as it offers the ability to have a multi-view perspective available in real-time (Montiel & Uyheng, 2022) and provide new and accurate insights (Thatcher et al., 2016). The buzz around big data has prompted new attention to previously overlooked communities but also exacerbates preexisting data disparities, particularly giving rise to a new social justice issue: data colonialism.
The movement for big data has also brought about a new manifestation of coloniality – data colonialism. The everyday collection of data surrounding our daily lives has naturally been converted into an ever-flowing stream of information, fundamentally creating a new social order (Herther, 2022). This social order consists of nonstop tracking and surveillance which brings with it unprecedented opportunities for prejudice, discrimination, and colonialism at large. While historic settler colonialism appropriated land and resources for settler profit and gain, data colonialism normalizes the appropriation and exploitation of communities through data (Herther, 2022; Couldry & Mejias, 2019). Data produced from data colonialism perpetuates policies and programs that further marginalize underrepresented knowledge systems and communities (Mills, 2022). Big data can be implicated within data colonialism by decontextualizing data from specific communities (Montiel & Uyheng, 2022). This decontextualization excludes community-driven knowledge, particularly Indigenous ways of knowing and being, and only works to amplify existing coloniality and perpetuate harm (Mills, 2022; Montiel & Uyheng, 2022).
The Cycle of Erasure
Indigenous communities are routinely left out of quantitative data reports, contributing to a cyclical issue in terms of representation and erasure. The cycle begins with the omission of American Indian and Alaska Native communities from racial/ethnic breakdowns in data. Most often, Indigenous populations are relegated into the “Other” racial category – representing an amalgamation of every other racial and ethnic minority community that was deemed too small of a sample to be included. This phenomenon has become so normalized that the National Congress on American Indians describes this as the Asterisk Nation (NCAI, n.d.), as Native data is represented by an asterisk instead of a data point. Ultimately, these “Other” racial and ethnic categories are rendered meaningless as there is no way to meaningfully distinguish between which groups are more or less included within this singular category.
The “Other” racial category, or the Asterisk Nation, also only exists when Native communities are included in data samples at all. More often than not, Indigenous participants are not intentionally sought out and sampled or constitute such a small amount that researchers omit their participation altogether. There is a false narrative that Native communities are among the most difficult to research due to geographic isolation and rurality, lack of familiarity with how to account for tribal affiliation, or overall general invisibility of our people. In reality, these are merely excuses for researchers to overlook Indigenous populations as the majority of Native people reside in urban areas, not reservations. Further, there is plenty of guidance available on proper accounting for tribal affiliation and cultural proximity. Finally, continuing to undersample or overlook Indigenous communities within quantitative data is yet another manifestation of the cycle of erasure.
The cycle then continues as Native data samples fail to meet statistical assumptions to run multivariate analyses as they require a sample size of n=100 at minimum. This then furthers erasure in the scientific literature as the majority of peer-reviewed publications will not even consider manuscripts that only include descriptive statistics. This then contributes to additional hurdles for scholars seeking to tell stories with Indigenous quantitative data. Lessened publications further feed into the overall lag of understanding of Native Nations through quantitative data and continue to perpetuate this ongoing erasure. The cycle begins all over again when scholars seek to build on previous work with Native samples as they will soon find a lack of representative data, publications, and general information on quantitative work with Indigenous communities overall. The full cycle can be seen below in Figure 1.
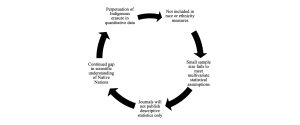
The Representation Paradox
Whenever Indigenous communities are represented within quantitative data, there is a unique issue surrounding generalizability. Quantitative measures tend to be overly inclusive as to who “counts” as American Indian or Alaska Native, resulting in an ethnic gloss. This essentially glosses over the diverse experiences, cultures, and nations into one conglomerate generic group which may not be truly representative of any specific Native Nation. Ethnic gloss is emblematic of the general lack of cultural specificity scholars have in working with Indigenous populations. This overall notion of a singular, monolithic Indigenous community/culture is referred to as pan-indigenous or pan-indigeneity. In reality, there are more than 574 federally recognized Native Nations in the United States, each with our own different cultures, histories, experiences, geographies, economies, etc. National generalizability through ethnic gloss purports to be generalizable to the “average Native” but lacks specificity to any Native Nation.
Conversely, scholars can choose to work specifically within tribal nations. While these results cannot generalize to all Indigenous communities nationally, it may be possible to extrapolate findings to similar tribes based on size, geography, and economy. However, in order to protect confidentiality of respondents, it may be difficult or unethical to explicitly name Native nations. Scholars must take great care to be sensitive to Indigenous communities’ history of harm in the name of research and work to respect tribal cultural protocols and confidentiality.
Ultimately, there is no one way to fully account for Native communities as we are a multitude of cultures and histories often grouped together into a singular Indigenous conglomerate. This issue of generalizability is a representation paradox – achieving either tribal specificity or national generalizability but not both. This paradox is often weaponized against Indigenous communities as yet another excuse as to why scholars fail to recognize or represent Native respondents in data.
The Movement to Decolonize Data
Decolonization is the undoing of colonialism whereby a nation re-establishes itself, including the dismantling of structures of oppression, reclaiming traditional ways of knowing and being, and addressing our own internalized colonization. The movement to decolonize data de-centers dominant western approaches by centering Indigenous knowledges and epistemologies to offer methodological possibilities to achieve racial and social justice, recognize tribal sovereignty, and ultimately move towards collective liberation. Decolonization has been applied to a myriad of fields, with big data being no exception. Scholars have begun the call to decolonize data.
The first step to decolonizing data is to name the practice of data colonialism (Couldry & Mejias, 2019), fundamentally rejecting the normalization of non-stop data extraction from our daily lives. While decolonizing data does not mean the total rejection of data collection in totality, rather it seeks to reject the appropriation and exploitative social order that has been brought about through the big data movement (Couldry & Mejias, 2019).
Decolonizing data is more than rejection and resistance but also recognition and renewal (Montiel & Uyheng, 2022). Recognition through decolonial data amplifies the voices of historically marginalized communities, such as Indigenous populations, owning that we can tell our own stories with our own data on our own terms. The decolonial act of renewals encourages new directions that resist coloniality and push for new horizons in the broader data-fied world around us (Montiel & Uyheng, 2022). Reclaiming and telling our own stories through data is a remedy to data colonialism, as the saying goes: Nothing about us, without us.
In order to remedy the cycle of erasure and representation paradox, guidance from the Urban Indian Health Institute on decolonizing data provides solutions. According to UIHI, best practices for data collection on American Indian and Alaska Native communities include mandating the collection of race and ethnicity in health data, collecting tribal affiliation, and intentionally oversampling Native populations (UIHI, n.d.). Rather than relying on the large Native conglomerate, collecting race and ethnicity in health data ensures that Indigenous communities have the opportunity to be represented. In collecting tribal affiliation, individuals can specify how they identify without impeding on tribal sovereignty or relying on verification of citizenship – which has historically been interrupted and attacked through colonial measures of blood quantum and interference from outside governments. Finally, oversampling as an intentional sampling process allows greater representation and the ability to adjust the population distribution of data overall (UIHI, n.d.). Each of these strategies are approaches to decolonizing data and supporting the movement for Indigenous data sovereignty.
Ultimately, all efforts to decolonize data must support Indigenous Data Sovereignty (IDS). Defined as the right of Indigenous people to control data from our communities and lands in both an individual and collective sense, including rights to access and privacy, Indigenous Data Sovereignty is a fundamental and inherent right of Native Nations. Indigenous data governance is defined as the entitlement to determine how Indigenous data is governed and stewarded (Rainie et al., 2019). Data governance under IDS includes the right to data generation of Indigenous peoples to support nation rebuilding and governance (Rainie et al., 2019). In order to better advocate for our communities, advance scientific understanding, and move towards identifying solutions, data practices around Indigenous communities must be decolonized. By centering Indigenous Data Sovereignty and supporting Indigenous data governance, scholars and practitioners alike can interrupt data colonialism and ultimately move towards decolonial futures.
References
Andersen, L. B., Christensen, M., Danholt, P., & Lauritsen, P. (2022). The role of digital data on citizens in social work research: A literature review. The British Journal of Social Work. https://doi.org/10.1093/bjsw/bcac158
Couldry, N., & Mejias, U. A. (2019). Data colonialism: Rethinking big data’s relation to the contemporary subject. Television & New Media, 20(4), 336–349. https://doi-org.du.idm.oclc.org/10.1177/1527476418796632
Coulton, C., Goerge, R., Putnam-Hornstein, E., & de Haan, B. (2015). Harnessing big data for social good: A grand challenge for social work. American Academy of Social Work and Social Welfare. Working Paper No. 11.
Herther, N. K. (2022). Data colonialism: A new way to look at the complex world of information. Information Today, 39(3), 16–19. http://newsbreaks.infotoday.com/NewsBreaks/Data-Colonialism-A-New-Way-to-Look-at-the-Complex-World-of-Information-151302.asp
Mills, D. (2022). Decolonial perspectives on global higher education: Disassembling data infrastructures, reassembling the field. Oxford Review of Education, 48(4), 474–491. https://doi.org/10.1080/03054985.2022.2072285
Montiel, C. J., & Uyheng, J. (2022). Foundations for a decolonial big data psychology. Journal of Social Issues, 78(2), 278–297. https://doi.org/10.1111/josi.12439
National Congress on American Indians (n.d.). Data. https://www.ncai.org/policy-research-center/research-data/data
Rainie, S., Kukutai, T., Walter, M., Figueroa-Rodriguez, O., Walker, J., & Axelsson, P. (2019) Issues in Open Data – Indigenous Data Sovereignty. In T. Davies, S. Walker, M. Rubinstein, & F. Perini (Eds.), The State of Open Data: Histories and Horizons. Cape Town and Ottawa: African Minds and International Development Research Centre.
Thatcher, J., O’Sullivan, D., & Mahmoudi, D. (2016). Data colonialism through accumulation by dispossession: New metaphors for daily data. Environment & Planning D: Society & Space, 34(6), 990–1006. https://doi.org/10.1177/0263775816633195
Urban Indian Health Institute (n.d.). Best Practices for American Indian and Alaska Native Data Collection. https://uihi.org