17
Markus Christen
Introduction: Scientific Virtues and Digitalization
The emergence of science and scientific thinking in modern Europe[1] has been accompanied by the development of a set of virtues that intend to characterize “good science” and “veritable scientists.” Also denoted as “epistemic virtues,”[2] they are preached and practiced in order to know the world; “they are norms that are internalized and enforced by appeal to ethical values, as well as to pragmatic efficacy in securing knowledge.”[3] Scientific virtues are not stable entities across time and not clearly separable from other kinds of virtues, which leads to the initial question in this essay: which scientific virtues should be of interest? For example, should we refer to “high-level virtues” such as a well-developed phronêsis, one of Aristotle’s four cardinal virtues? Certainly, this virtue is also critical to practicing science; in order to produce sound scientific knowledge, scientists must be able to deliberate well about their work and the work of others.[4] However, phronêsis as virtue covers a broad spectrum of human activities and is thus beyond the scope of this short essay.
Instead, should we refer to a very fine-grained virtue ontology and focus on a broad set of virtue candidates? Darcia Narvaez, Timothy Reilly, and colleagues created an impressive list of virtues (broadly construed)[5] relevant for scientific inquiry, including caution (showing appropriate caution with respect to various contingencies), collegiality (working with and for colleagues), foresight (planning ahead and foreseeing possibilities), imagination (visualizing or conceptualizing abstract entities), open-mindedness (being receptive to new ideas or information, especially that which goes against conventional wisdom), and recognition (appreciating and valuing the contribution of others to your work). Certainly, all those virtues refer to important demands for science (and for other types of human collaborative endeavors)—but assessing such a long list would again exceed the aims of this short essay.
Instead, I suggest focusing on six scientific virtues proposed by Pennock and O’Rourke[6] and emerging from Pennock’s “Scientific Virtues Project.” These virtues directly refer to the basic scientific goal of discovering empirical truths about the natural world. Pursuing this goal requires distinctive traits that a scientist should cultivate; because of science’s special aims, curiosity and intellectual honesty are the primary scientific virtues. Other virtues play important related roles. Pennock and O’Rourke mention skepticism and objectivity as important scientific virtues. Moreover, as repeatable empirical testing is not easy, especially when one must quantify results, perseverance and meticulousness are valuable qualities for scientists.
As the aim of this essay is to assess the impact of changing scientific practices on scientific virtues due to technological developments, my second question is: What influences the practice of science? This is obviously a broadly discussed theme both within the history of science and the philosophy of science on the nature and the causes of scientific change. Answering this question requires a reference to both macro-scale sociological factors (work pioneered by scholars such as Joseph Ben-David[7]) as well as micro-level, that is, the concrete work of scientists in experimental systems (see for example the work of Hans-Jörg Rheinberger.)[8] The ongoing digital transformation likely impacts both the macro- and micro-scale of scientific activity, as the second section will outline. Based on this short general sketch of the nature of the current digital transformation, the third section will speculate on the possible impact of the use of digital tools on these six virtues. A short conclusion outlines potential positive and negative uses of new digital tools in the scientific practice with reference to the cultivation of scientific virtues.
Digitalization: The Next Wave Driven by Machine Learning
Using digital tools in science is certainly not a new phenomenon. Beginning from the theoretical and practical foundation of modern computation in the 1930s and 1940s, computers became an indispensable tool for many scientific disciplines; they enabled “big science” and allowed for the emergence of new fields that strongly relied on computer simulations.[9] On the broader societal level, the application of information technology is not new, and resulting phenomena such as the automation of production processes are well-studied.[10] However, the key differences between today’s digital transformation and the previous use of computer technology result from the combination of advances in the field of machine learning (ML), enormously increased data availability, and greatly increased computing power. ML-generated artificial intelligence (AI) systems increasingly solve problems where traditional computer programs fail. In contrast to explicitly written programs, new types of AI systems (so-called deep learning algorithms) are trained by being exposed to a multitude of examples and rewarded for making the right decisions. Their learning imitates human learning to some degree, although the latter includes emotional engagement and purpose. Within a few years, these advances have enabled AI systems to achieve impressive success in demanding and ambiguous tasks such as image recognition, translation, radiological image analysis, and gaming. They not only compete with human abilities, but also sometimes even surpass them. These techniques are improving rapidly and lead to applications that were previously reserved only for people, such as driving vehicles or diagnosing illnesses. AI thus becomes an enabling technology for an enormous range of applications.
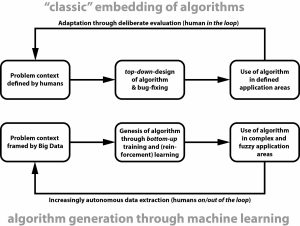
The associated change is profound because the way algorithms are embedded in social systems is fundamentally changing, as Figure 1 illustrates. Until recently, people have explicitly defined the algorithmically manageable problems, created the necessary programs top-down, and applied the algorithms in clearly distinguishable areas. But now, large amounts of diverse data form the (often only incompletely understood) basis of the problem context, machine learning generates the problem-solving algorithm, which is then used in increasingly heterogenic and blurred application areas, whereby the applications act increasingly autonomously and exchange information with each other. Thus, the “feedback” between concrete problem solving by means of an algorithm in a defined area of application and the resulting adaptation of the definition of the problem is increasingly happening with limited or even without human control. The result is a gradual shift from decision support through algorithms to the automation of decisions in areas relevant to life, such as lending, mobility, legal examinations, or access to resources. Therefore, the public discussion about the application of AI is often dominated by dystopian future scenarios.[11]
Artificial intelligence is a branch of computer science that deals with the automation of intelligent behavior using concepts from other disciplines such as neuroscience and cognitive science. Since the concept of intelligence itself is relatively diverse, there is no clear scientific definition of AI. The origins of AI go back to the 1950s, and this initial phase was marked by almost limitless expectations about the capability of computers. This attitude was regularly criticized, and the high expectations raised by “old AI” have so far not been fulfilled. However, current technical innovations have changed this assessment to a certain degree. Machine learning has become the basic technology for self-driving cars, robot assistants, and the automation of non-trivial social, administrative, and economic processes. Technological progress, the training of qualified practitioners, and competitive pressure are accelerating the spread of AI.
Accordingly, various discourses have developed in recent years. These are briefly described below because they provide orientation points for assessing the potential impact of AI and Big Data on scientific practice:
The black box problem: In contrast to “classic” computer algorithms, the new ML technologies—especially the so-called deep neural networks—use different programming techniques. Instead of clear software structures, which are at least comprehensible in principle for the programmer, a neural network is provided by the programmer, but its connectivity and weighting of the connections change over an enormous number of training cycles (an image recognition algorithm is trained with millions of images, for example). In the end, even the developers do not know how the algorithm comes to the solution, because such ML models are equations that have no obvious physical or logical basis. Therefore, certain AI algorithms appear as “black boxes,” a significant limitation for practical applications of AI, provided there is an expectation that one understands how a system comes to a decision.[12] When using AI for automated translation, the problem is probably irrelevant, especially since determining the quality of a translation is simple, but if the system is to decide on a customer’s creditworthiness, for example, both customers and users need to know what criteria the system uses to make its decisions.
The bias problem: As Figure 1 illustrates, (big) data is the central resource for AI algorithms; this is particularly true in the case of deep learning. Depending on the type of decision problem, however, one-sidedness or biases can be hidden in the data, which then shape the behavior of the algorithm.[13] A well-known example is that the Google search for “professional hair” returns mostly images of white women, while the search for “unprofessional hair” shows primarily black women. This classification reflects the bias hidden in the data. AI systems can even be manipulated and thus abused by means of inappropriate learning data. In March 2016, a Microsoft experiment that ran a Twitter account using artificial intelligence failed. The fictitious AI teenager began to tweet increasingly racist and misogynistic statements after being deliberately influenced by a group of Twitter users. The bias problem is relevant because the user is unlikely to be able to identify hidden one-sidedness in training data sets consisting of a million units.
The fairness problem: Important questions arise not only with regard to the data, but also with regard to the algorithms themselves, because these contain implicit normative assumptions and are thus value-laden. Important parameters are defined by the developers and configured intentionally or unintentionally by the users in such a way that certain values and interests are privileged over others. This is relevant if AI systems are used, for example, to assess criminals. The problem of the fairness of algorithms is complex, because given legal norms must be translated into a “language” that computer programs can understand. For example, algorithms can be constructed in such a way that they systematically ignore certain data characteristics (for example, information on gender or social status), but this often influences the accuracy of the algorithm.[14] Other intuitions of fairness can be “algorithmically packaged” in such a way that, for example, the proportion of classification errors of the first and second order (X is falsely assigned or falsely not assigned to group Y) may not be differentiated across discrimination-relevant groups.[15] However, mathematical considerations show that certain ethically equally justifiable demands on algorithms (for example, regarding accuracy and fairness) cannot be met simultaneously.[16] Thus, the designers are forced to make moral choices when creating algorithms.
The problem of trust: In view of the problems described above, a paradoxical finding of psychological research is that people apparently tend to trust the results of automated decision-making too much. This manifests a basic problem of ML models that reveal correlation when it cannot be known whether or not they reveal causation. There is a risk that decisions depending on these models will be made with an illusion of security, even though they are only based on alleged connections that are not causally secure. A study from Stanford, for example, shows that participants rated the discriminatory employment recommendation of an algorithm as better and more neutral than the same recommendation made by a human.[17] However, the reverse problem (algorithm aversion) is also known: Studies show, for example, that evidence-based algorithms predict the future of certain types of problems more accurately than human forecasters do. But when people have to decide whether to use a human prognosticator or a statistical algorithm, they often choose the former—even when they see that the latter exceeds human capability.[18] This is apparently because people lose confidence in algorithmic procedures faster than in human forecasters. These studies point to a complex problem of trust when people increasingly rely on automated decisions: there is evidence of both too much and too little trust. This may indicate that a social practice for dealing with automated decisions has yet to be established.
Economic effects: The economic consequences of digital change clearly occupy the largest place in social discourse—and AI has a key role here in view of the enormously broad application potential. In contrast to previous automation pushes, activities that previously seemed to be reserved for people can now potentially be replaced. Some studies have predicted that up to 50 percent of all occupations could be automated in the next twenty years[19] and even highly qualified work will not be spared. Even though the extent of job losses is highly controversial[20] and the potential for creating new jobs is unclear, hardly any occupational field of AI should remain unaffected, including science. Computers and the internet have already redefined entire industries such as media, music, and travel; more are likely to follow. And even if such economic upheavals have already taken place several times, they have always been accompanied by social unrest and crises. Given that modern information technologies have produced considerable wealth for a rather small group of well-trained people and enormous wealth for a very small group of the privileged,[21] the potential for social unrest is undoubtedly there.
The monopoly problem: Another economic problem complex concerns the relevant players in the research and development of AI systems. Since the new forms of ML are strongly data-based, companies with access to enormously large data sets have a competitive advantage. Leading technology companies from China and the United States such as Alibaba, Amazon, Baidu, Facebook, Google, and Microsoft are redesigning their internal business processes and products around AI. The well-known “winner takes all” effect of the internet economy and the associated danger of monopoly formation is likely to intensify in view of the large resources required for the development of successful AI systems. This could make science increasingly dependent on large tech companies.
Geostrategic issues: A final, important point concerns geostrategic issues. China has defined AI as a key element in its strategic goal of becoming a global leader in the development of new technologies. At the same time, AI is a powerful instrument for supporting totalitarian efforts such as mass surveillance of the population and “big nudging.” In 2020, China plans to introduce a nationwide social credit system based on comprehensive monitoring and assessment of citizens by AI systems.[22] The question is to what extent the national application of AI technologies developed in societies with divergent social norms and democratic traditions raises ethical or political problems. Military uses of AI also fall within this complex of topics, and scientists are already warning of an “AI arms race.”[23] This is a dynamic that is difficult to understand and even more difficult to control.
This is an impressive list of issues related to the current digital transformation powered by AI and Big Data—and they raise many questions far beyond this short essay. Nevertheless, let us take this list as a framework for assessing the potential impact of using digital tools in science.
Digitalization of Science Impact Assessment: What Can We Expect?
We have to be aware of the pitfalls of the current discourse on the digital transformation of science and society. Some claims are exaggerated and partly driven by the economic interests of either the tech industry or the consultancy industry. Nevertheless, digital transformation is an ongoing process that will likely affect the practice of science in many ways. Some recent examples illustrate this:
- The ability to analyze large, unstructured data sets will increase tremendously. Unlike earlier attempts, “deep learning” systems do not need to be programmed with a human expert’s knowledge. Instead, they learn on their own, often from large training data sets, until they can see patterns and spot anomalies in data sets that are far larger and messier than human beings can cope with. This can be used to greatly decrease the time needed in discovery processes, for example when analyzing complex chemical reactions.[24]
- Digital tools will also allow new ways of visualizing large data sets, including interactive visualizations and embedded simulation tools to make immediate predictions.[25]
- A startup called iris.ai creates exploration tools, starting from free-text description of the problem and a result editor, to build a large corpus of documents related to the problem statement with the aim of generating a precise reading list. In the long term, they want to build an “AI scientist” that can create a hypothesis based on existing publications, run experiments and simulations, and even publish papers on the results.[26]
- This goal of a “robot scientist” has already been realized in genetics: At the University of Wales at Aberystwyth, Ross King’s program “Adam” designed and ran genetics experiments. Its successor “Eve,” at the University of Manchester, is designed to automate early-stage drug development: drug screening, hit conformation, and cycles of hypothesis learning and testing.[27]
- A team at IBM and colleagues has created a system that can generate scientific hypotheses automatically by mining academic literature. Moreover, their algorithms, they say, can be used to make new scientific discoveries. Their goal is to combine text mining with visualization and analytics to identify facts and suggest hypotheses that are “new, interesting, testable and likely to be true,”[28]
- The system “Science Surveyor” uses algorithms to characterize the scientific literature on a selected topic. Using the abstract and citations of a peer-reviewed paper, Science Surveyor provides journalists context about that paper in several easy-to-read visualizations.[29]
These examples show that digital support is or will be available for various aspects of scientific work such as:
- Deciding what to read through systems that assess the importance of published scientific work
- Deciding which scientific question to assess through systems that are able to systematically explore the “problem space” for interesting spots.
- Creating hypotheses through systems that can survey what has been published so far.
- Deciding on the originality of research questions through systems that gain semantic understanding of what already has been explored (one could imagine some kind of higher-level “plagiarism engine.”)
- Actually performing the experiments, or at least the repetitive, “boring” parts of some experiments.
- Perform deductive reasoning based on the results generated in the experiments.
- Writing the papers (at least some sections with a high degree of standardization, such as the methodology section) or ensuring that the text written by scientists is “machine readable” (that it will be legible to the systems that automatically “read” them after publication and keep track of the scientific literature body).
- Reviewing papers through systems that may assess novelty of findings or find shortcomings in the argumentation or even data fraud.
- Deciding who would be a good collaboration partner through reputation systems that evaluate the “match” of scientists or teams.
This list is not conclusive; all aspects of scientific practice can be shaped at least partly by digital tools. However, as practicing science is the way scientific virtues are trained and shaped, I ask, how will these digital tools affect those virtues? In the following, I will provide a (speculative) assessment of the six virtues proposed by Pennock and O’Rourke using an evaluation framework that is based on the issues of the general AI and big data discourse mentioned in the previous section.
How Virtues May be Affected by AI in Science
The first relevant scientific virtue is curiosity. Curious scientists want to discover something. They want to find the answer to a question or they want to test whether some hypothesis is true. They have the drive to find new interesting questions. In short, they want to know something about the world. Although AI-supported digital tools lack the intrinsic motivation of generating knowledge about the world,[30] they may indeed be used by scientists to explore a problem space systematically in a way the single scientist never can do by himself or herself. One may say that curiosity is “externalized” from the scientist to such a system; the scientist then would be less involved in the process of finding questions, but is presented with questions that result from an externalized problem space exploration. As finding new interesting questions is a competitive advantage in today’s science funding and career system, curiosity as a virtue might be hampered, particularly in scientific fields, where the availability of data allows for the construction of problem spaces. The problem of bias then could become particularly relevant, as an incomplete problem space (whose incompleteness remains undiscovered) could make relevant questions inaccessible for the AI system. Depending on what algorithms are used, the black box issue may have some relevance (because the scientist does not necessarily see why the system believes a certain question is promising); and in this way, the trust problem is intensified. As the data sets need to be large for creating the problem space, in some fields the monopoly problem—that is, the dependence of scientists on the data of large platform providers—could be relevant. Finally, AI-driven problem-space exploration may also have the effect that creative thinkers would not be attracted to science any longer.
The second key virtue is intellectual honesty, honesty in the acquisition, analysis, and transmission of scientific ideas, theories, or models. The vices corresponding to this virtue, such as deliberately ignoring facts, falsifying data, or plagiarism, are recognized as major problems for scientific advancement. Here, AI systems, perhaps used in the peer review process, indeed have the potential to support intellectual honesty by identifying scientists who infringe against this virtue. Used in this way as a control instrument, however, the digital tools would not directly enhance intellectual honesty; they would be instruments to detect “sinners.” An obvious issue here is fairness, as it may be opaque why a control system qualifies a certain scientist as intellectually dishonest. Some tools may be used by intellectually honest scientists themselves, to check whether a seemingly new idea is indeed original. However, whether using such tools in the process of becoming a scientist indeed supports intellectual honesty might be questionable: the repeated experience that one’s own ideas are evaluated to be “not original” by such systems (which is a likely scenario for students) may have unintended effects, creating frustration and even the motivation to “trick” such systems.
The virtue of meticulousness might be most strongly affected by automating some aspects in (experimental) science that are repetitive and “boring,” but such “boring” parts of practicing science might be exactly what is needed to develop meticulousness. Thus, this virtue might eventually be externalized to the machine. Furthermore, the issue of economic effects may come into play here, as AI support systems may replace human workers in those repetitive or monotonous tasks where young scientists get their first involvement in the actual practice of science.
The virtue of objectivity involves a lack of bias or prejudice when making scientific judgments as well as the ability to make decisions based on facts rather than on personal feelings or beliefs. Digital tools that would be used for hypothesis finding, deductive reasoning, or assessment of scientific publications are likely to have a “flavor of objectivity,” and their use by scientists may indeed be motivated by this virtue. Surely, the issues of bias (in the data) and fairness (of the algorithms) may come into play here, depending on the concrete applications. A more interesting problem, however, could be that the use of digital tools to increase objectivity may lead to an “exaggeration” of this virtue by downplaying the diversity of ideas that is likely important for scientific progress. If a well-constructed AI system relying on a large scientific database “decides” that a certain question is the relevant one—who could argue against that? The use of AI tools may create high standards of objectivity that undermine the chances to be wrong and learn through those mistakes.
The virtue of perseverance is important in a scientific culture where people know that progress is slow and that many ideas do not work, and where frustration due to failure is common. The various ways digital tools can be used indeed have the potential to make scientific practice much more efficient (inducing the economic effects already mentioned)—and perseverance may lose much of today’s importance in the scientific domains where those tools allow for substantial efficiency gains. Whether this will actually be the case is hard to say, because one could imagine that a new type of perseverance might become relevant—the perseverance necessary to make the digital tools work the way they are expected to work. People dealing with complex software need a lot of patience until they really understand their tools; the same might happen with the digital tools intended to make scientific practice more efficient. However, people would then spend less time with the object of scientific inquiry and more time with their support tools.
Finally, the virtue of skepticism could be affected by those tools as well, maybe as a side effect of “over-enhanced” objectivity and the use of such tools as control instruments to detect intellectual dishonesty. Obviously, the issue of trust comes into play here; whether the problem is too much or too little trust will depend on the concrete application. One aspect to consider here, however, concerns the geostrategic implications of a widespread promotion of AI applications for pursuing political goals (“big nudging,” mass surveillance, and so forth.) Skepticism (and non-conformist behavior) is often the target of such goals, and tools for evaluating the reputation of the work of a scientist may be turned against the skeptical scientist.
Conclusion
This brief outline is sketchy and needs more reflection, but it makes clear that there is reason to believe that digital tools for scientists emerging from the progress in big data analytics and AI will likely affect scientific virtues. The perspective here is rather critical with respect to the impact of AI on those virtues. To what extent these potential dangers will be realized certainly remains an open question—on the one hand, because the promises of the “new AI” may (once again) go unfulfilled, as the usefulness of AI for complex scientific tasks remains limited. In such a scenario, AI systems would be just one tool out of many, and thus not this technology, but other factors related to the social conditions in which science is performed will likely have a stronger impact on scientific virtues.
On the other hand, the rise of AI in scientific practice is not an inevitable and deterministic phenomenon. Human considerations do play a role and they have an impact on how we train and use machine learning applied to various social domains.[31] The possibility that future scientists may have “AI companions” supporting their research in various ways is not restricted to a purely instrumental and uniform worldview. Depending on the use of this technology, it may also enhance pluralism in thinking. If AI systems in the far future may indeed gain a degree of autonomy with respect to the scientific ideas they suggest or the honesty they demand, they may remind us that scientific practice can also be holistic and respectfully, relationally attuned to the natural world and to the autonomy of other-than-humans.[32] But ensuring that the digitalization of science fosters scientific virtues demands a reflective use of the digital tools that will likely change scientific practice tremendously.
MARKUS CHRISTEN is a Research Group Leader at the Institute of Biomedical Ethics and History of Medicine and Managing Director of the UZH Digital Society Initiative. He received his M.Sc. in philosophy, physics, mathematics, and biology at the University of Berne, his Ph.D. in neuroinformatics at the Federal Institute of Technology in Zurich, and his habilitation in bioethics at the University of Zurich. He researches in empirical ethics, neuroethics, ICT ethics, and data analysis methodologies. He has over 100 publications in the fields of ethics, complexity science, and neuroscience.
Bibliography
- Altmann, Jürgen, and Frank Sauer. “Autonomous Weapon Systems and Strategic Stability.” Survival 59.5 (2017): 117–42.
- Ben-David, Joseph. Scientific Growth: Essays on the Social Organization and Ethos of Science. Berkeley: University of California Press, 1991.
- Berk, Richard, Hoda Heidari, Shahin Jabbari, Matthew Joseph, Michael Kearns, Jamie Morgenstern, Seth Neel and Aaron Roth. “A Convex Framework for Fair Regression.” June 9, 2017. https://arxiv.org/abs/1706.02409v1.
- Brynjolfsson, Erik, and Andrew McAfee. The Second Machine Age: Work, Progress, and Prosperity in a Time of Brilliant Technologies. New York: W.W. Norton, 2014.
- Caliskan, Aylin, Joanna J. Bryson, and Arvind Narayanan. “Semantics Derived Automatically from Language Corpora Contain Human-like Biases.” Science 356 (2017): 183–86.
- Chen, Jiin-Yu. “Virtue and the Scientist: Using Virtue Ethics to Examine Science’s Ethical and Moral Challenges.” Science and Engineering Ethics 21 (2015): 75–94.
- Christen, Markus, Darcia Narvaez, and Eveline Gutzwiller. “Comparing and Integrating Biological and Cultural Moral Progress.” Ethical Theory and Moral Practice 20 (2016): 55.
- Christen, Markus, Nikola Biller-Andorno, Berit Bringedal, Kevin Grimes, Julina Savulescu, and Henrik Walter. “Ethical Challenges of Simulation-Driven Big Neuroscience.” AJOB Neuroscience 7.1 (2016): 5–17.
- Daston, Lorraine, and Peter Galison. Objectivity. New York: Zone Books, 2007.
- Dietvorst, Berkeley J., Joseph P. Simmons, and Cade Massey. “Algorithm Aversion: People Erroneously Avoid Algorithms After Seeing Them Err.” Journal of Experimental Psychology: General 144.1 (2015): 114–26.
- Frey, Carl Benedict, and Michael A. Osborne. “The Future of Employment: How Susceptible are Jobs to Computerisation?” September 7, 2013. https://www.oxfordmartin.ox.ac.uk/downloads/academic/The_Future_of_Employment.pdf.
- Hardt, Moritz, Eric Price, and Nathan Srebro. “Equality of Opportunity in Supervised Learning.” October 7, 2016. https://arxiv.org/abs/1610.02413.
- Henning, Klaus, and Maike Süthoff, eds. Mensch und Automatisierung: Eine Bestandesaufnahme. Opladen: Westdeutscher Verlag, 1990.
- Kleinberg, Jon, Sendhil Mullainathan, and Manish Raghavan. “Inherent Trade-Offs in the Fair Determination of Risk Scores.” November 17, 2016. https://arxiv.org/abs/1609.05807v2.
- Loi, Michele, and Markus Christen. “How to Include Ethics in Machine Learning Research.” ERCIM News 116 (2019): 5.
- Pasquale, Frank. The Black Box Society: The Secret Algorithms that Control Money and Information. Cambridge, MA: Harvard University Press, 2015.
- Pennock, Robert T., and Michael O’Rourke. “Developing a Scientific Virtue-Based Approach to Science Ethics Training.” Science and Engineering Ethics 23 (2017): 243–62.
- Rheinberger, Hans-Jörg. Toward a History of Epistemic Things. Stanford: Stanford University Press, 1997.
- Rossi, Paolo. Die Geburt der Modernen Wissenschaft in Europa. München: Beck-Verlag, 1997.
- Ulissi, Zachary W., Andrew J. Medford, Thomas Bligaard, and Jens K. Nørskov. “To Address Surface Reaction Network Complexity Using Scaling Relations Machine Learning and DFT Calculations.” Nature Communications 8 (2017). https://doi.org/10.1038/ncomms14621
- Williams, Kevin, Elizabeth Bilsland, Andrew Sparkes, Wayne Aubrey, Michael Young, Larisa N. Soldatova, Kurt De Grave, Jan Ramon, Michaela de Clare, Worachart Sirawaraporn, Stephen G. Oliver, and Ross D. King. “Cheaper Faster Drug Development Validated by the Repositioning of Drugs Against Neglected Tropical Diseases.” Journal of the Royal Society–Interface 12 (2015). https://doi.org/10.1098/rsif.2014.1289
- Paolo Rossi, Die Geburt der Modernen Wissenschaft in Europa (München: Beck-Verlag, 1997). ↵
- Lorraine Daston and Peter Galison, Objectivity (New York: Zone Books, 2007). ↵
- Ibid., 40. ↵
- Jiin-Yu Chen, “Virtue and the Scientist: Using Virtue Ethics to Examine Science’s Ethical and Moral Challenges,” Science and Engineering Ethics 21 (2015): 75–94. ↵
- Personal communication during the “Developing Virtues in the Practice of Science” project. ↵
- Robert T. Pennock and Michael O’Rourke, “Developing a Scientific Virtue-Based Approach to Science Ethics Training,” Science and Engineering Ethics 23 (2017): 243–62. ↵
- Joseph Ben-David, Scientific Growth: Essays on the Social Organization and Ethos of Science (Berkeley: University of California Press, 1991). ↵
- Hans-Jörg Rheinberger, Toward a History of Epistemic Things (Stanford, CA: Stanford University Press, 1997). ↵
- Markus Christen, Nikola Biller-Andorno, Berit Bringedal, Kevin Grimes, Julina Savulescu and Henrik Walter, “Ethical Challenges of Simulation-Driven Big Neuroscience,” AJOB Neuroscience 7.1 (2016): 5–17. ↵
- Klaus Henning and Maike Süthoff, eds., Mensch und Automatisierung: Eine Bestandesaufnahme (Opladen: Westdeutscher Verlag, 1990). ↵
- For an overview see https://en.wikipedia.org/wiki/Existential_risk_from_artificial_general_intelligence. ↵
- Frank Pasquale, The Black Box Society: The Secret Algorithms That Control Money and Information (Cambridge, MA: Harvard University Press, 2015). ↵
- Aylin Caliskan, Joanna J. Bryson, and Arvind Narayanan, “Semantics Derived Automatically from Language Corpora Contain Human-like Biases,” Science 356 (2017): 183–86. ↵
- Moritz Hardt, Eric Price, and Nathan Srebro, “Equality of Opportunity in Supervised Learning” (2016), https://arxiv.org/abs/1610.02413. ↵
- Richard Berk, Hoda Heidari, Shahin Jabbari, Matthew Joseph, Michael Kearns, Jamie Morgenstern, Seth Neel, and Aaron Roth, “A Convex Framework for Fair Regression” (2017), https://arxiv.org/abs/1706.02409v1. ↵
- Jon Kleinberg, Sendhil Mullainathan, and Manish Raghavan, “Inherent Trade-Offs in the Fair Determination of Risk Scores” (2016), https://arxiv.org/abs/1609.05807v2. ↵
- Arthur Jago, “Technology and (in)discrimination,” paper presented at Psychology of Technology Conference, Berkeley, CA, 2017, available at https://www.psychoftech.org/2017-schedule. ↵
- Berkeley J. Dietvorst, Joseph P. Simmons, and Cade Massey, “Algorithm Aversion: People Erroneously Avoid Algorithms After Seeing Them Err,” Journal of Experimental Psychology: General 144.1 (2015): 114–26. ↵
- Carl Benedict Frey and Michael A. Osborne, “The Future of Employment: How Susceptible are Jobs to Computerisation?,” working paper, 2013, https://www.oxfordmartin.ox.ac.uk/downloads/academic/The_Future_of_Employment.pdf. ↵
- Max Rauner, “Die Pi-mal-Daumen-Studie,” Zeit Online, 2017, http://www.zeit.de/2017/11/kuenstliche-intelligenz-arbeitsmarkt-jobs-roboter-arbeitsplaetze. ↵
- Erik Brynjolfsson and Andrew McAfee, The Second Machine Age: Work, Progress, and Prosperity in a Time of Brilliant Technologies (New York: W.W. Norton, 2014). ↵
- Felix Lee, “Die AAA-Bürger,” Zeit Online, 2017, http://www.zeit.de/digital/datenschutz/2017-11/china-social-credit-system-buergerbewertung. ↵
- Jürgen Altmann and Frank Sauer, “Autonomous Weapon Systems and Strategic Stability,” Survival 59.5 (2017): 117–42. ↵
- Zachary W. Ulissi, Andrew J. Medford, Thomas Bligaard, and Jens K. Nørskov, "To Address Surface Reaction Network Complexity Using Scaling Relations Machine Learning and DFT Calculations," Nature Communications 8 (2017), https://doi.org/10.1038/ncomms14621. ↵
- For an illustration, see the work and publications of the University of Washington Interactive Data Lab: http://idl.cs.washington.edu/. ↵
- See https://iris.ai/. ↵
- Kevin Williams, Elizabeth Bilsland, Andrew Sparkes, Wayne Aubrey, Michael Young, Larisa N. Soldatova, Kurt De Grave, Jan Ramon, Michaela de Clare, Worachart Sirawaraporn, Stephen G. Oliver, and Ross D. King, "Cheaper Faster Drug Development Validated by the Repositioning of Drugs Against Neglected Tropical Diseases," Journal of the Royal Society–Interface 12 (2015), https://doi.org/10.1098/rsif.2014.1289. ↵
- Scott Spangler, Angela D. Wilkins, Benjamin J. Bachman, Meena Nagarajan, Tajhal Dayaram, Peter Haas, Sam Regenbogen, Curtis R. Pickering, Austin Comer, Jeffrey N. Myers, Ioana Stanoi, Linda Kato, Ana Lelescu, Jacques J. Labrie, Neha Parikh, Andreas Martin Lisewski, Lawrence Donehower, Ying Chen, and Olivier Lichtarge, "Automated Hypothesis Generation Based on Mining Scientific Literature," paper presented at KDD 2014, New York, NY, August 24–27, 2014, http://dx.doi.org/10.1145/2623330.2623667. ↵
- See https://science-surveyor.github.io/. ↵
- In the following, I do not discuss the speculation that AI may lead to a (self-conscious) “super-intelligence,” which may develop such intrinsic motivations. ↵
- Michele Loi and Markus Christen, “How to Include Ethics in Machine Learning Research,” ERCIM News 116 (2019), 5. ↵
- Markus Christen, Darcia Narvaez, and Eveline Gutzwiller, “Comparing and Integrating Biological and Cultural Moral Progress,” Ethical Theory and Moral Practice 20 (2016): 55. ↵