Marco Madella
Marco Madellaabc
a CaSEs, Department of Humanities, Universitat Pompeu Fabra, C/Trias Fargas 25-27, 08005 Barcelona, Spain. Associated Unit to IMF-CSIC.
b ICREA, Passeig Lluís Companys 23, 08010 Barcelona, Spain.
c School of Geography, Archaeology and Environmental Studies, The University of the Witwatersrand, Johannesburg, South Africa.
SimulPast aimed at developing an innovative and interdisciplinary methodological framework to model and simulate ancient societies, and their relationship with environmental transformations. In order to achieve this, the project attempted to define “best practices” for simulation in the Humanities and Social Sciences and to consolidate a new research line in the use of formal modelling and simulation to investigate past human societies and, as a result, to support a better understanding of the present.
Computer modelling and complex systems simulation dominated the scientific debate over the last two decades, providing important outcomes in biology, geology and life sciences, and resulting in the origin of entirely new disciplines such as bioinformatics or geoinformatics. However, ten years ago most social scientists continued to consider simulation as a less interesting tool for their investigations as the perception was that reproducing “inside a computer” what past societies did and believed was not possible, because of the perceived complexity of social structures.
The teams involved in SimulPast considered that this skepticism was largely due to anthropocentrism. Human behaviour may be complex, however many other systems being studied by scientists are as intricate as social structures. Furthermore artificial intelligence has shown how the appropriate interconnection of very simple computational mechanisms is able to show extraordinary complex patterns, and now that access to distributed supercomputing (grid technologies) is affordable, it is no longer possible to justify not applying these methods to the Humanities and Social Sciences.
The origin of SimulPast was the realisation of a group of researchers that progress in the acquisition of better, more precise and more reliable data was not being matched with corresponding progress in the analysis of such data and the conclusions that could be drawn. Building on their knowledge of other fields and on the initiatives that were at the time underway in their own domain, we decided to unite our efforts in order to investigate a model and simulation-based analytical approach.
Social and environmental transitions represent key aspects to better understand human behaviour. From a complex systems perspective, the most decisive questions about human societal systems are related to the transitions between phases of equilibrium. Therefore, the study of these transitions is extremely interesting in order to move forward with our current understanding of human behaviour at macro, meso and micro-level. In that respect, ancient societies present a great opportunity to build a virtual laboratory in which to model, explore and simulate different hypotheses and theories about social and environmental transitions.
Archaeology is particularly well suited for modelling and simulation. It is a data oriented subject, with a strong focus on the collection of objective material data for the study of past human societies. Furthermore, it is a discipline with a long tradition of attempting to bridge the divide between the Social Sciences and Humanities on one side and the Natural and Formal Sciences on the other. Finally, it deals with events in the past and therefore provides the possibility of comparing the results of the run experiments with the facts that are known to have occurred.
The project intended to demonstrate the applicability of models and that these models were able to provide answers, which would not be obtainable using other methodological approaches. A model also acts as a “neutral” backdrop to express phenomena and ideas in a common way that can be understood by researchers from the Social Sciences and Humanities as well as the Formal and Natural Sciences. Moreover, because models can describe changes occurring in complex sets of relationships, they are extremely useful to formalize dynamic theories that can be rigorously tested against observed data.
Simulation expresses a theory and this theory produces a series of empirical predictions that can be directly contrasted with the real world. It thus makes it possible to check assumptions and correct or reformulate theories. In the Social Sciences and Humanities this provides a unique opportunity to develop and validate new techniques and new approaches, not only with a view to support the investigation of the past but also to provide a better understanding of the present. SimulPast has used simulation as a virtual laboratory in which different techniques have been exploited to formalize and falsify scientific hypotheses about social transformations. Different case studies integrating empirical data collection and general theories acted in certain cases as validating opportunities for the model(s).
Traditionally modelling and simulation techniques are split between two different perspectives: a) ecologically influenced or b) oriented to artificial societies. The first perspective is mostly interested in environmental constraints and resource exploitation and management, often neglecting the importance of human behaviour and cultural constraints that intervene in this process. The second perspective makes use of simplified virtual societies to investigate theoretical questions, thus removing what should be a basic component of the model: the environment where human interactions take place. SimulPast has strived to unify these two perspectives. It is essential to model complex behaviour, and simulate the decision-making processes of people, but in addition it is imperative to consider the constraints defined by the environment, which may affect these very same actions. Therefore, our project has been focusing on models that explored the spatio-temporal evolution of concrete socio-ecological units, as well as their size and distribution on complex stratified landscapes (geological, ecological, socio-political, etc.). Furthermore, the project’s teams were also convinced that abstract simulation models could significantly aid the development of a more formal approach to the model-building process. For that reason SimulPast investigated abstract simulation models to explore emergent properties and complex adaptive systems. Our diverse case studies were the starting points to contrast precise, small-scale socio-ecological questions as well as to validate and to corroborate more general theories. We considered that the constant exchange between these two perspectives provided an exemplar methodological paradigm and consolidated an innovative proposal for simulation in the Social Sciences and Humanities.
The critical mass that SimulPast generated (with 63 researchers and PhD students involved from the start of the project plus the new personnel hired specifically by the project, for a grand total at one point of 85 researchers) has been a unique example of a “transversal” project testing the potential for tight collaboration between the Human and Social Sciences (Archaeologists, Anthropologists and Sociologists) and the Formal Sciences (Mathematicians, Logicians and Computer Scientists).
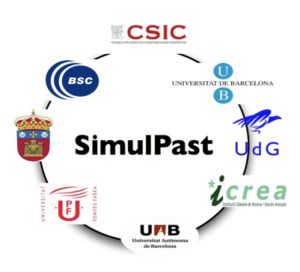
1. Background and development
SimulPast was developed around five theoretical, analytical and practical areas that were expressed as work packages in the project’s organization (Figure 2):
- WP1 – Data structure/model and problem formulation
- WP2 – Modelling
- WP3 – Simulation
- WP4 – Verification and validation
- WP5 – Dissemination
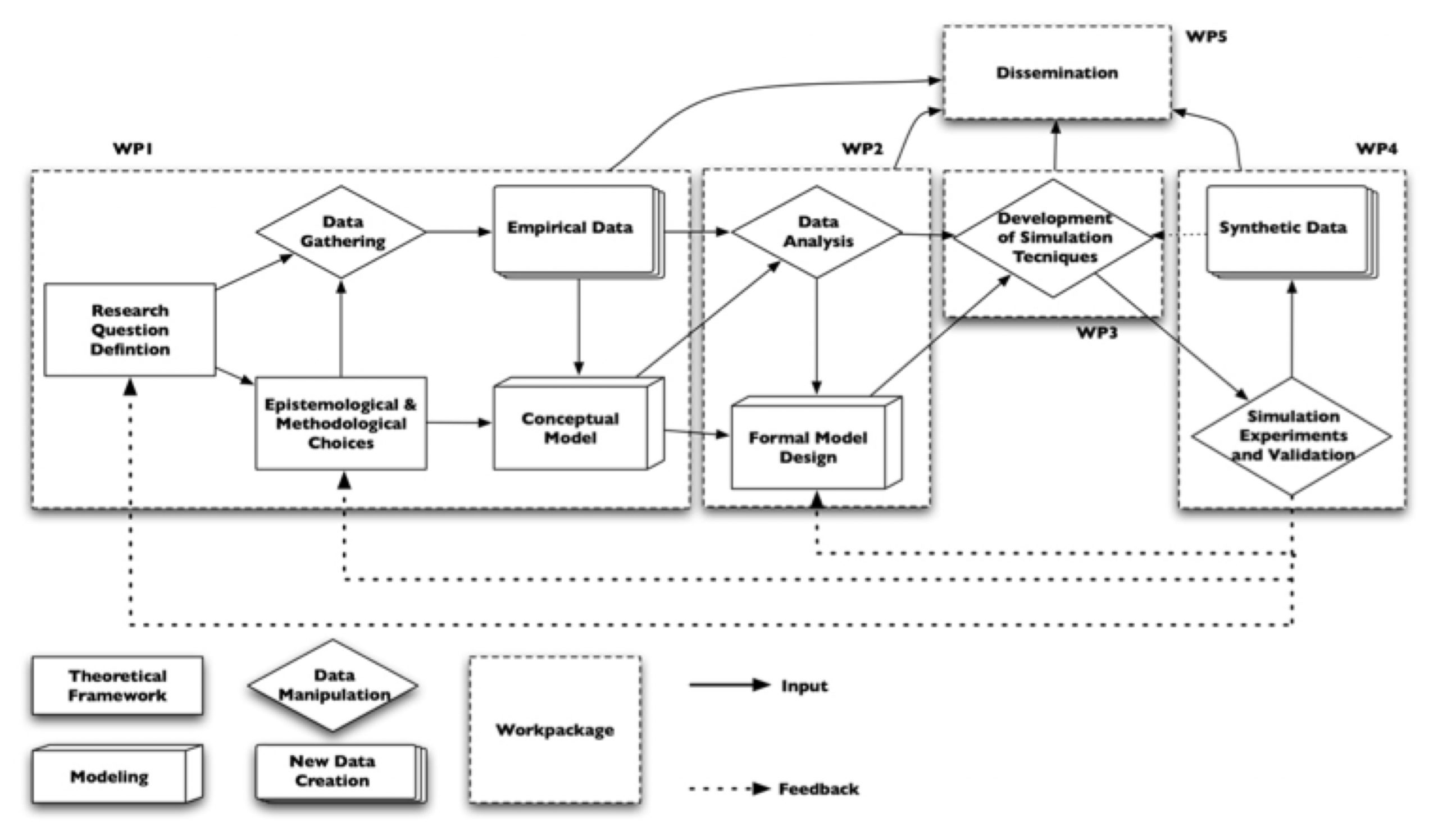
The first step of the project was the definition of a series of specific research problems related to past human behaviour and approached through different case studies (Figure 3).
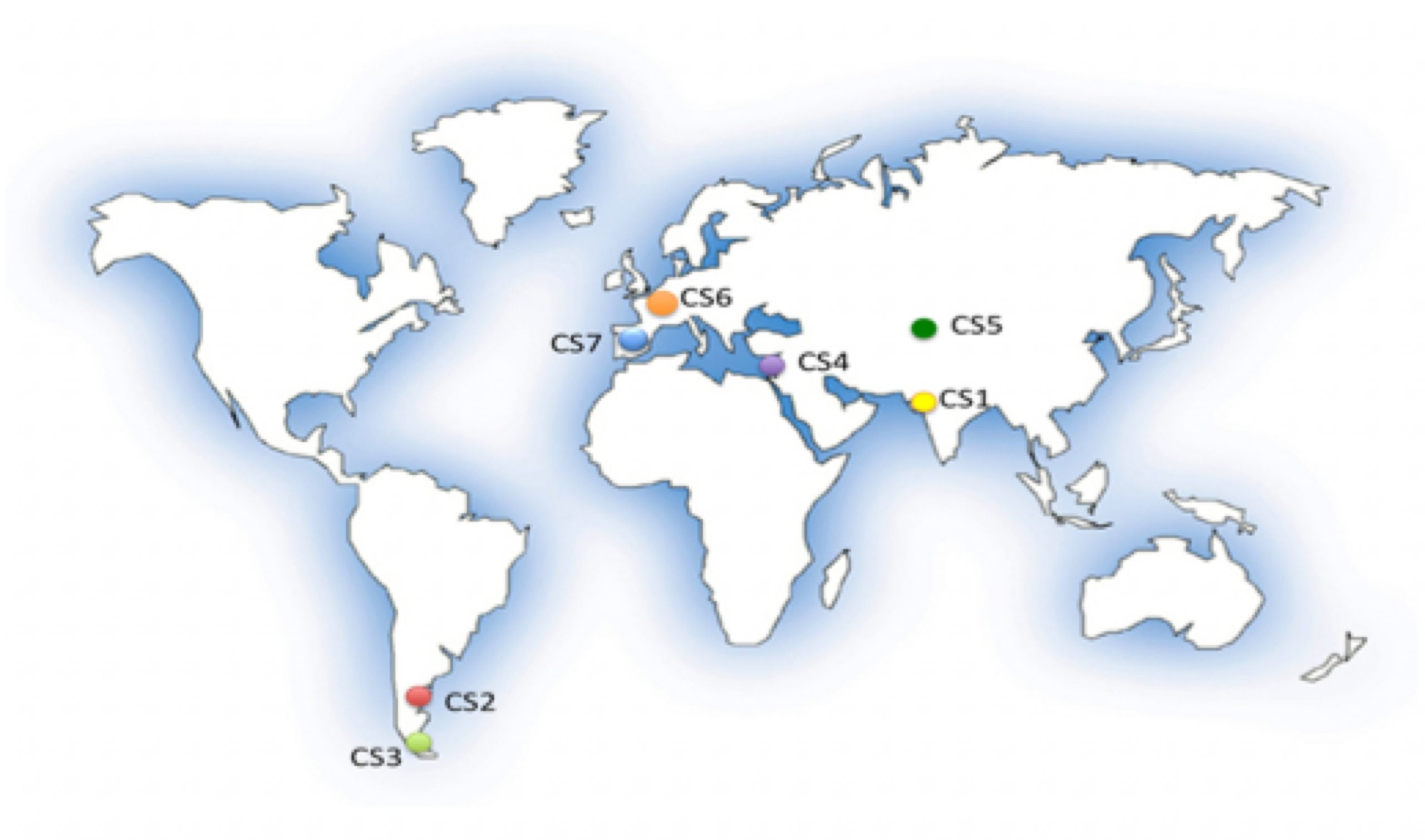
CS1: Resources, seasonality and landscape in the Holocene of northern Gujarat (India).
CS2: Emergence of ethnicity in hunter-gatherer societies in Patagonia (Argentina).
CS3: Social cooperation and conflict in late hunter-gatherer societies of Tierra del Fuego (Argentina).
CS4: Exchange networks dynamics in early the near eastern societies (Syria).
CS5: Adaptive systems for resource exploitation in Central Asia (Uzbekistan).
CS6: Origin and spread of agriculture in prehistoric Europe.
CS7: Dynamics of social interactions and adoption of innovation in prehistoric Europe.
These case studies served to test the three non-linear aspects of human societies that were the focus of SimulPast:
- Adaptive systems and resource exploitation strategy (CS1 and CS3).
- Emergent properties and social dynamics (CS2, CS4 and CS6).
- Networking and complex interactions (CS5 and CS7).
To facilitate the exchange of data between the diverse groups it was necessary to use a formally controlled process to standardize the encoding and description of the archaeological and simulation data. This process is known as data modelling. In general, four levels of abstraction can be recognized within data modelling:
- The first of these is observation of reality, which is the range of phenomena we wish to model as they actually exist or are perceived to exist in all their complexity.
- The second level is the conceptual model, which is the first stage abstraction and incorporates only those parts of reality considered to be relevant to the particular application. A cartographic map is a good metaphor for the conceptual model as a map only contains those features that the cartographer has chosen to represent and all other aspects of reality are omitted. This provides an immediate simplification, though a sense of reality can still be readily interpreted or reconstituted from it.
- The third level is the logical model, often called the data structure. This is a further abstraction of the conceptual model into lists, arrays, and matrices that represent how the features of the conceptual model are going to be entered and viewed in the database, handled within the code of the software, and prepared for storage. The logical model can generally be interpreted as reality only with the assistance of software, such as by creating a display.
- The fourth level is the file structure (sometimes called physical structure). This is the final abstraction and represents the way in which the data are physically stored on the hardware or media as bits and bytes.
1.1- Modelling
A model of a thing is a representation of such thing in a different context. A model may have more or less characteristics in common with the object: a model railroad is fairly close to a real railroad except in scale, a ship in a bottle is a reproduction of the outward appearance of a ship, but in a completely different functional context. The word “model” describes ways of thinking about a certain subject or problem — as in “Adam Smith’s model of the market”, meaning an understanding of supply, demand and competition in terms of an “invisible hand”, or “the entity/relationship model”, meaning the approach to think about reality in terms of entities and relations between them.
A traditional approach to modelling in the Natural Sciences is the formulation of a problem as a set of complete and consistent mathematical descriptions, which give some insight into the problem the researcher is trying to solve. This, as with all modelling techniques, focuses on the search for answers to the original research questions, simplifying the problem through the elimination of all the unimportant and unrelated topics around them. If the model is correctly designed, solving the equations will improve the understanding of the problem and, hopefully, validate or invalidate the working hypothesis.
In contrast, the use of models within Engineering has followed a different approach, representing aspects of the real world in an abstract form suitable for computer processing – a technique commonly called conceptual data modelling. The conceptual model for a simulation addresses the simulation’s context, how it will satisfy its requirements, and how its entities and processes will be represented. The conceptual model is key to assessing a simulation’s validity for any situation not explicitly tested and determining the appropriateness of a simulation (or its parts) for reuse or use with other simulations in a distributed simulation. It is important to note that there are no widely accepted approaches for decomposing the representation of the simulation subject into the entities and processes of a simulation’s conceptual model, for abstracting such representation from available information about the subject, or for describing and documenting the simulation’s conceptual model.
The search for valid models within the Humanities and Social Sciences has been more problematic. It is considered difficult to create systems able to meaningfully represent human behaviour and interaction (both with the environment as well as other humans). This is the reason why most models remain formulated as verbal constructs or mechanical analogies (e.g. market laws). The major drawbacks of verbal models are the ambiguity of human language and the practicality of validation. In contrast to mathematical modelling, a particular verbal model will raise strong discussion about every concept within it, as it will have different meanings for different researchers, and it will be difficult to explore through objective computer simulation or mathematical analysis. The solution is provided by the definition of a formal model, clean of ambiguities and closer to a mathematical/computer model, and thus capable of being implemented as a simulation. There exist different approaches to this problem (graphs and networks, unified modelling language, ontologies, game theory, logical representation, etc.), dependent on the discipline. Unfortunately, these are often not flexible enough to be useful in problems that encompass widely different research areas or which have not been tested in different knowledge domains.
SimulPast has given special emphasis on designing a common formal modelling framework, useful and understandable for all the groups involved. This was possible thanks to the expertise of the project partners in formal modelling from a Computer Science perspective (G9 and G10), but also because the four teams from the Humanities and Social Sciences have been previously working on the development of formal models (G4, G6, G8 and G11).
1.2- Simulation
Simulation is akin to an experimental methodology. It is possible to set up a simulation model and then execute it many times, and by varying the conditions in which it is run it is possible to explore the effects of the diverse parameters.
Statistical models (for example, a set of equations) are based on an abstraction from the presumed social processes and the use of data with which to perform the estimation (for example, survey data on the variables included in the equation). The analysis consists of two steps: first, the researcher asks whether the model generates predictions that have some similarity to the data that have actually been collected (this is typically assessed by means of tests of statistical hypotheses); second, the researcher measures and compares the magnitude of the parameters, in order to identify the most significant.
Simulation also involves the development of a model based on hypotheses and supposed social processes. However, while strong resemblances between statistical models and simulation models exist, there are also important differences. In simulation, the model takes the form of a computer program rather than a statistical equation. The simulated data can then be compared with the data collected from the case studies (WP1) to check for similarity/dissimilarity between the prediction of the model and the reality.
Simulation models are concerned with processes, whereas statistical models typically aim to explain correlations between variables measured at one single point in time. We would expect a simulation model to include explicit representations of the processes that are thought to be at work in the social world. In contrast, a statistical model will reproduce the pattern of correlations among measured variables, but will rarely model the mechanisms that underlie these relationships. Nonlinear systems are difficult to study using statistical methods because there is often no set of equations that can be solved to predict the characteristics of the system. The only effective way of exploring nonlinear behaviour is therefore to simulate it by building a model and then running simulations.
The earliest use of simulation techniques in the Social Sciences dates back to the 1960s. Such early work was not widely accepted because most research tried to create predictive models in a deterministic way, an approach that wasn’t suited to studies where human behaviour played a key role. Moreover, the lack of objective quantitative data forced the researchers to rely on debatable assumptions, and the methodological framework was weak.
Simulation became a strong research trend within Social Sciences starting from the 1990’s (Gilbert & Troitzsch 2005). This was linked with the emergence of mathematical and computing disciplines such as artificial intelligence (Russell & Norvig, 2004), complex systems (Mitchell, 2009) and chaos theory (Kiel & Elliott, 1996). All of these help the researcher to explore and understand the dynamics of systems where the basic entities execute processes of decision-making and interaction with other entities. This mechanism can produce a chain of events capable of modifying the system and enabling new behavioural patterns to emerge from a bottom-up perspective (Schelling, 2006), often with chaotic qualities (small variations in the original state can heavily alter the outcomes).
These improved capabilities to model complex and chaotic systems through the addition of decision-making processes are the main reasons why the new simulation tools are better suited to design social science models, than the classical equation-based approach used in other research fields. They explain the rapid increase in the application of these techniques over the last 15 years, with the creation of dedicated scientific journals (e.g. Journal of Artificial Societies and Social Simulation, Artificial Life MIT), the organization of international conferences (e.g. Winter Simulation Conference, World Congress Social Simulation) and the establishment of specialised research centres (e.g. Santa Fe Institute in the USA, Centre for the Evolution of Cultural Diversity in the UK).
In archaeology, the use of complex systems and computer simulation to analyse past events has followed a parallel trend (for an early example see Doran & Palmer, 1995). A particularly important development has been the integration of archaeological data and landscape reconstruction using Geographical Information Systems in a perspective of computer simulation (for a general view see Barceló, 2009, Bentley & Maschner, 2003). The G6 and G7 SimulPast groups were at the forefront of this process, which is embedded in the structure of the project as a whole.
SimulPast focused on three specific non-linear aspects:
- Adaptive systems and resource exploitation strategy
- Emergent properties and social dynamics
- Networking and complex interactions
Therefore, we concentrated in the development and application of four major simulation techniques in support of this focus, and to best utilise the available expertise and data:
- Agent-Based Modelling (ABM)
- Probabilistic Reasoning and Statistical Learning
- Complex network analysis
- Partial differential equations and integro-difference equations
1.3- Verification and Validation
Once the results have been collected we need to translate this (sometimes large) volume of data into information suitable to be used in order to verify the entire process and validate hypotheses.
Verification – sometimes called “internal validation”, e.g. by Taylor (1983), Axelrod (1997), Drogoul et al., (2003), and Sansores & Pavón (2005), or “program validation”, e.g. by Stanislaw (1986) and Richiardi et al., (2006) – is the process of ensuring that the model performs in the manner intended by its designers and implementers (Moss et al., 1997).
The process of verification and validation is particularly crucial when using computer models for scientific purposes. As argued above, computer simulation offers the potential to enhance the transparency, soundness, descriptive accuracy and precision of the modelling process, but it aggravates the difficulty of the verification process. Computer simulation models (and especially agent-based models) are generally complex and mathematically intractable, so making sure that there is no significant disparity between what we think the computer code is doing and what it is actually doing – i.e. verifying the model – is of utmost importance and can be surprisingly challenging:
“You should assume that, no matter how carefully you have designed and built your simulation, it will contain bugs (codes that do something different to what you wanted and expected)” (Gilbert, 2008).
“An unreplicated simulation is an untrustworthy simulation – do not rely on their results, they are almost certainly wrong (‘wrong’ in the sense that, at least in some detail or other, the implementation differs from what was intended or assumed by the modeller)” (Edmonds & Hales, 2003).
“Achieving internal validity is harder than it might seem. The problem is knowing whether an unexpected result is a reflection of a mistake in the programming, or a surprising consequence of the model itself. […] As is often the case, confirming that the model was correctly programmed was substantially more work than programming the model in the first place” (Axelrod, 1997).
1.4- Dissemination
The activities related to dissemination carried out during the 78 months of the project have been many and diverse but they can be generally grouped into internal and external dissemination.
Internal
- The Internal Workshops were conceived as focused moments of discussion among the SimulPast members and they were related to specific work packages, or to specific developmental steps in the project.
- The Internal Reading Groups that aimed at promoting interdisciplinary debate on a specific theme, including the ongoing results of the project. A published work or theme was selected for discussion before each reading group meeting, which was then chaired by the person who suggested the reading/theme.
- The Seminars were conceived as working meetings for SimulPast members with the aim of exchanging knowledge and opinions, and included discussion of working papers, of transversal topics, definition of a common language, invited talks and lectures, or technical tutorials. Within the Seminars are also listed the Training Events normally organised by SimulPast members/groups but open to both project members and external people.
External
- The External Workshops were organised or co-organised by SimulPast members but also had the participation of external researchers and in some cases explored parallel research topics of interest for the project. In this category are also listed the organization of sessions in or full international conferences by one or more groups of the project.
- Conference talks, lectures and posters were used to disseminate and discuss with the wider public the results of our research during the various stages of the project.
- The Milestone Publications, these represent the activity related to the publication of project’s results related to specific goals, milestones or achievements. The publications are normally in the form of a journal Special Issue or a book.
- The General Publications, these represent all the publications arising from the SimulPast dynamics. They might not have been linked to specific original goals and/or milestones but they were nonetheless originating from the project’s research environment.
SimulPast personnel published a total of 293 papers, book chapters, conference proceedings and other various publications arising from the project work. This production averages 3.75 publications for each month of the project.
2. Research advances from the SimulPast
The project made advances in a series of methodological challenges that were open or greatly debated within the context of computer simulation in social sciences:
1. The use of computer simulation for theory building versus hypothesis testing, or in other words, exploratory versus explanatory models.
a. The project has managed to explore and develop simulation supporting theory building as well as hypothesis testing showing the adaptability of such an approach.
2. Realistic versus abstract simulation.
a. SimulPast explored these two realms of simulation approaches and it has been able to make inroads in assessing viability and strength of each one while developing the project’s case studies.
3. Model-based versus rule-based. Is the introduction of complex decision-making algorithms and cognitive models useful (and computationally feasible) for understanding cultural processes?
a. The project has been able to develop comparative research on these approaches and made substantial developments on the use of cognitive models.These contributions were crystallized around a methodological debate and three thematic clusters (cultural transmission, resilience, cooperation), which resulted in specific milestone outcomes (Figure 4).
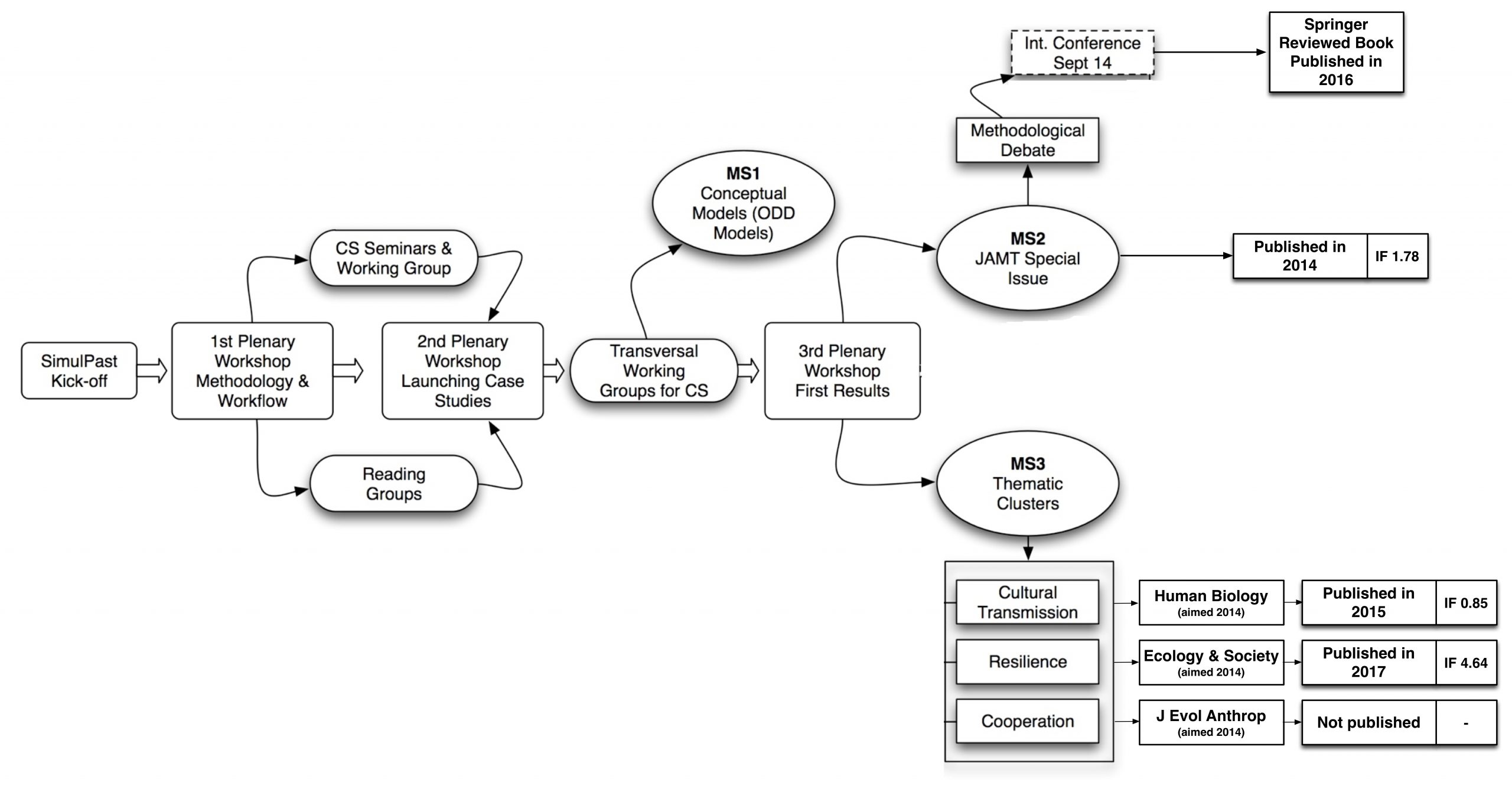
Furthermore, more general outcomes were:
- A better and deeper understanding of the processes of social transformation.
- Despite the steep increase of the application of computer simulation in many fields over the last 40 years, its impact in archaeology had been minimal. SimulPast increased the availability of simple software environments together with publication of models implemented in “accessible” languages. This played a role in changing this situation by widening the community with access to this tool.
And practical ones:
- Development and validation of new software for social simulation:
- Pandora, an HPC Agent-Based Modelling framework (https://www.bsc.es/computer-applications/pandora-hpc-agent-based-modelling-framework) The impact of this development can be assess in the number of publications and communications based on the used of the library (28 papers/communications – https://www.bsc.es/computer-applications/pandora/publications)
- Cassandra (complementing Pandora), a program developed to analyse the results generated by simulations created with the library. Cassandra allows the user to visualize the complete execution of simulations using a combination of 2D and 3D graphics, as well as statistical figures.
Bibliography
Axelrod, R. (1997). Advancing the art of simulation in the social sciences. (R. Conte, R. Hegselmann, & P. Terna) Complexity, 21-40. Springer-Verlag.
Barceló, J.A. (2009). Computational intelligence in archaeology. Information Science Reference.
Bentley, R., & Maschner, H. (2003). Complex systems and archaeology: empirical and theoretical applications. University of Utah Press.
Doran, J., & Palmer, M. (1995). The EOS project: Integrating two models of palaeolithic social change. (N. Gilbert & R. Conte) Artificial societies: The computer simulation of social, pp. 103-125.
Drogoul, A., Vanbergue, D., & Meurisse, T. (2003). Multi-agent based simulation: where are the agents? (J. S. Sichman, F. Bousquet, & P. Davidsson). Multi-agent-based simulation II.
Edmonds, B., & Hales, D. (2003). Replication, replication and replication: Some hard lessons from model alignment. Journal of Artificial Societies and Social, 6.
Gilbert, G., & Troitzsch, K. (2005). Simulation for the social scientist. Springer-Verlag.
Gilbert, N. (2008). Agent-based models (quantitative applications in the social sciences). Springer-Verlag.
Kiel, L., & Elliott, E. (1996). Chaos theory in the social sciences: Foundations and applications. University of Michigan, USA.
Mitchell, M. (2009). Complexity. A guided tour. Oxford University Press.
Moss, S., Edmonds, B., & Wallis, S. (1997). Validation and Verification of Computational Models with Multiple Cognitive Agents. Manchester Metropolitan University, Centre for Policy Modelling.
Richiardi, M., Leombruni, R., Saam, N., & Sonnessa, M. (2006). A Common Protocol for Agent-Based Social Simulation. Journal of Artificial Societies and Social Simulation, 9.
Russell, S. & Norvig, P. (2004). Inteligencia Artificial – Un Enfoque Moderno. Pearson – Prentice Hall.
Sansores, C., & Pavón, J. (2005). Agent-based simulation replication: A model driven architecture approach. In MICAI 2005: Advances in Artificial Intelligence, 4th Mexican International Conference on Artificial Intelligence, Monterrey, Mexico, November 14-18, 2005, Proceedings. Lecture Notes in Computer Science, pp. 244-253.
Schelling, T. C. (2006). Micromotives and Macrobehavior. W. W. Norton.
Stanislaw, H. (1986). Tests of computer simulation validity. What do they measure? Simulation and Games, 17, pp. 173-191.
Taylor, A. (1983). The Verification of Dynamic Simulation Models. Journal of the Operational Research Society, 34, pp. 233-242.