7.2 Causal relationships
Learning Objectives
- Define and provide examples of idiographic and nomothetic causal relationships
- Describe the role of causality in quantitative research as compared to qualitative research
- Identify, define, and describe each of the main criteria for nomothetic causal relationships
- Describe the difference between and provide examples of independent, dependent, and control variables
- Define hypothesis, be able to state a clear hypothesis, and discuss the respective roles of quantitative and qualitative research when it comes to hypotheses
Most social scientific studies attempt to provide some kind of causal explanation. A study on an intervention to prevent child abuse is trying to draw a connection between the intervention and changes in child abuse. Causality refers to the idea that one event, behavior, or belief will result in the occurrence of another subsequent event, behavior, or belief. In other words, it is about cause and effect. It seems simple, but you may be surprised to learn there is more than one way to explain how one thing causes another. How can that be? How could there be many ways to understand causality?
Think back to our chapter on paradigms, which were analytic lenses comprised of assumptions about the world. You’ll remember the positivist paradigm as the one that believes in objectivity and social constructionist paradigm as the one that believes in subjectivity. Both paradigms are correct, though incomplete, viewpoints on the social world and social science.
A researcher operating in the social constructionist paradigm would view truth as subjective. In causality, we would report what people tell us so that we can understand what caused what. That seems straightforward, right? Well, what if two different people saw the same event from the exact same viewpoint and came up with two totally different explanations about what caused what? A social constructionist would say that both people are correct. There is not one singular truth that is true for everyone, but many truths created and shared by people.
When social constructionists engage in science, they are trying to establish idiographic causality. An idiographic causal explanation means that you will attempt to explain or describe your phenomenon exhaustively, based on the subjective understandings of your participants. These explanations are bound with the narratives people create about their lives and experience, and are embedded in a cultural, historical, and environmental context. Idiographic causal explanations are so powerful because they convey a deep understanding of a phenomenon and its context. From a social constructionist perspective, the truth is messy. Idiographic research involves finding patterns and themes in the causal relationships established by your research participants.
If that doesn’t sound like what you normally think of as “science,” you’re not alone. Although the ideas behind idiographic research are quite old in philosophy, they were only applied to the sciences at the start of the last century. If we think of famous scientists like Newton or Darwin, they never saw truth as subjective. They posed laws of science that were objectively true and applicable in all situations. Newton, Darwin, and others operated within the positivist paradigm, which continues to dominate much of science today. When positivists try to establish causality, they try to come up with a broad, sweeping explanation that is universally true for all people. This is the hallmark of a nomothetic causal explanation.
Nomothetic causal explanations are also incredibly powerful because they allow scientists to make predictions about what will happen in the future, with a certain margin of error. Moreover, they allow scientists to generalize—that is, make claims about a large population based on a smaller sample of people or items. Generalizing is important, as we clearly do not have time to ask everyone their opinion on a topic, nor can we look at every interaction in the social world. We need a type of causal explanation that helps us predict and estimate truth in all situations.
If these still seem like obscure philosophy terms, let’s consider an example. Imagine you are working for a community-based non-profit agency serving people with disabilities. You are putting together a report to lobby the state government, and you need to support your argument for additional funding for further community support programs at your agency. By referring to nomothetic research, you would learn that previous studies have linked community-based programs like yours to positive health and employment outcomes for people with disabilities. Nomothetic research seeks to explain that community-based programs are better for everyone with disabilities. By referring to idiographic research, you would learn what it is like to be a person that is involved in a community-based program by reading their personal accounts of their lived experiences. Using idiographic research, you can understand what it’s like to be a person with a disability and then communicate that to the state government. For example, a person might say “I feel at home when I’m at this agency because they treat me like a family member” or “this is the agency that helped me get my first paycheck.”
Neither kind of causal explanation is better than the other. Deciding to conduct idiographic research means that you will attempt to explain or describe your phenomenon exhaustively while attending to cultural context and subjective interpretations. Deciding to conduct nomothetic research means that you will try to explain what is true for everyone and predict what will be true in the future. In short, idiographic explanations have greater depth, and nomothetic explanations have greater breadth. Most importantly, social workers understand the value of both approaches to understanding the social world. A social worker helping a client with substance abuse issues seeks idiographic knowledge when they ask about that client’s life story, investigate their unique physical environment, or probe how they understand their addiction. The social worker also utilizes nomothetic research to apply generalizable knowledge about what typically helps people with substance use disorders, such as minimizing risk factors, maximizing protective factors, and employing evidence-based therapy techniques.
Nomothetic causal relationships
One of my favorite classroom moments occurred early in my teaching career. I had instructed my students to form groups, discuss the research questions they had drafted for their projects, and provide feedback to each other. I overheard one group trying to help a student rephrase their research question. One student asked the other, “are you trying to generalize… or nah?” In their own vernacular, they were referring to nomothetic causal relationships.
So, are you trying to generalize your potential research findings… or nah? Answering this question can you understand how to conceptualize and design your research project. If you are trying to generalize, or create a nomothetic causal relationship, then the rest of these statements are likely to be true: you will use quantitative methods, reason deductively, and engage in explanatory research. How can I know all of that? Let’s take it part by part.
Nomothetic causal relationships aim to generalize. For phenomena to be generalizable, they must be precisely measured and reduced to universally understood terms, such as mathematics and statistics. On one hand, not all quantitative methods explanatory in nature. For example, a descriptive study could reveal the number of people without homes in your county, though it won’t tell you why they are homeless. On the other hand, nearly all explanatory studies are quantitative. Explanatory researchers want a clean “x causes y” explanation, so they utilize the universal language of mathematics to achieve their goal. In sum, nomothetic causal relationships use quantitative methods to achieve generalizability and prove cause and effect relationships.
When we talk about x and y, we are talking about the relationships between variables. When one variable causes or contributes to change in another, we have what researchers call independent and dependent variables. For our example on spanking and aggressive behavior, spanking would be the independent variable and aggressive behavior would be the dependent variable. An independent variable is the cause, and a dependent variable is the effect. Dependent variables depend on independent variables. If you find yourself confused, remember the graphical relationship below.
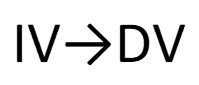
When attempting to make claims of a nomothetic causal relationship, it is important to consider the relationship’s strength. Relationship strength does not refer to the strength of your friendships or a marriage, rather in this context it refers to statistical significance. The more statistically significant a relationship between two variables is shown to be, the greater confidence we can have in the strength of that relationship. If you recall our discussion of statistical significance in Chapter 3, you’ll remember that it is usually represented as the p value in statistics.
A hypothesis is a statement that describes the researcher’s expectation regarding what they anticipate to find. In quantitative research, hypotheses are a nomothetic causal relationship that the researcher expects to demonstrate between the independent and dependent variables. Your prediction should be taken from a theory or model of the social world. For example, you may hypothesize that treating clinical clients with warmth and positive regard is likely to help them achieve their therapeutic goals. That hypothesis would be using the humanistic theories of Carl Rogers. Using previous theories to generate hypotheses is an example of deductive research. If Rogers’ theory of unconditional positive regard is accurate, your hypothesis should be true. In sum, all nomothetic causal relationships must use deductive reasoning.
Let’s consider a couple of examples. In research on sexual harassment (Uggen & Blackstone, 2004), [1] one might hypothesize, based on feminist theories of sexual harassment, that more females than males will experience specific sexually harassing behaviors. What is the causal relationship being predicted here? Which is the independent and which is the dependent variable? In this case, we hypothesized that a person’s gender (independent variable) would predict their likelihood to experience sexual harassment (dependent variable).
Sometimes researchers will hypothesize that a relationship will take a specific direction. As a result, an increase or decrease in one area might be said to cause an increase or decrease in another. For example, you might choose to study the relationship between age and support for legalization of marijuana. Perhaps you’ve taken a sociology class utilized theories you’ve read to hypothesize that age is negatively related to support for marijuana legalization. [2] You have just hypothesized that as people get older, the likelihood of their supporting marijuana legalization decreases. Thus, as age (your independent variable) moves in one direction (up), support for marijuana legalization (your dependent variable) moves in another direction (down). In addition, positive relationships involve two variables going in the same direction and negative relationships involve two variables going in opposite directions. If writing hypotheses feels tricky, it is sometimes helpful to draw them out and depict each of the two hypotheses we have just discussed.
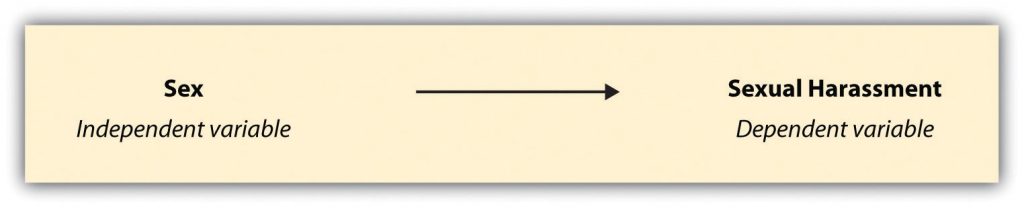
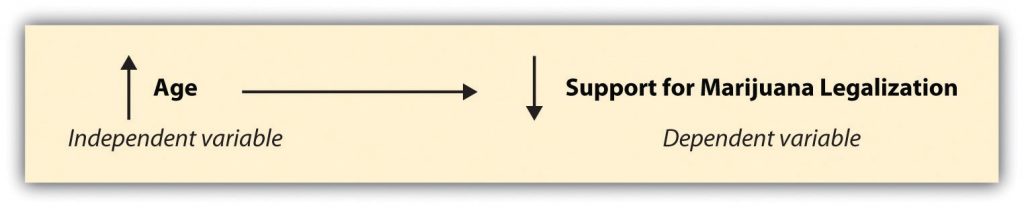
It’s important to note that once a study starts, it is unethical to change your hypothesis to match the data that you found. What happens if you conduct a study to test the hypothesis from Figure 7.3 on support for marijuana legalization, but you find no relationship between age and support for legalization? In this example, your hypothesis was wrong, but you can still draw valuable information from your incorrect prediction. Your study would challenge what the existing literature says on your topic and therefore demonstrate that more research needs to be done to figure out the factors that impact support for marijuana legalization. Don’t be embarrassed by negative results, and definitely don’t change your hypothesis to make it appear correct all along!
Let’s say you conduct your study and you find evidence that supports your hypothesis. As age increases, support for marijuana legalization decreases. Success! Causal explanation complete, right? Not quite. You’ve only established one of the criteria for causality. The main criteria for causality have to do with covariation, plausibility, temporality, and spuriousness. In our example from Figure 7.3, we have established only one criteria—covariation. When variables covary, they vary together. Both age and support for marijuana legalization vary in our study. Our sample contains people of varying ages and varying levels of support for marijuana legalization.
The presence of some correlation between two variables does not mean that a causal relationship between the two is plausible. Plausibility means that in order to make the claim that one event, behavior, or belief causes another, the claim has to make sense. It makes sense that people from previous generations would have different attitudes towards marijuana than younger generations. People who grew up in the time of Reefer Madness or the hippies may hold different views than those raised in an era of legalized medicinal and recreational use of marijuana.
Once we’ve established that there is a plausible relationship between the two variables, we also need to establish whether the cause happened before the effect, the criterion of temporality. A person’s age is a quality that appears long before any opinions on drug policy, so temporally the cause comes before the effect. It wouldn’t make any sense to say that support for marijuana legalization makes a person’s age increase. Even if you could predict someone’s age based on their support for marijuana legalization, you couldn’t say someone’s age was caused by their support for legalization.
Finally, scientists must establish nonspuriousness. A spurious relationship is one in which an association between two variables appears to be causal but could be explained by a third variable. For example, we could point to the fact that older cohorts are less likely to have used marijuana, which could influence their negative attitudes toward legalization. This is often referred to as the third variable problem, where a seemingly true causal relationship is actually caused by a third variable not included in the hypothesis. In this example, the relationship between age and support for legalization could be more about having tried marijuana than the age of the person.
Quantitative researchers are sensitive to the effects of potentially spurious relationships. They are an important form of critique of scientific work. As a result, they will often measure these third variables in their study, so they can control for their effects. These are called control variables, and they refer to variables whose effects are controlled for mathematically in the data analysis process. Control variables can be a bit confusing, but think about it as an argument between you, the researcher, and a critic.
Researcher: “The older a person is, the less likely they are to support marijuana legalization.”
Critic: “Actually, it’s more about whether a person has used marijuana before. That is what truly determines whether someone supports marijuana legalization.”
Researcher: “Well, I measured previous marijuana use in my study and mathematically controlled for its effects in my analysis. The relationship between age and support for marijuana legalization is still statistically significant and is the most important relationship here.”
Let’s consider a few additional, real-world examples of spuriousness. It could be argued, albeit incorrectly, that high rates of ice cream sales cause drowning because there is a positive relationship between the two variables. In this case, the time of year (summer) is the third variable that causes both high ice cream sales and increased deaths by drowning (Babbie, 2010). [4] Here’s another good one: it is true that as the salaries of Presbyterian ministers in Massachusetts rise, so too does the price of rum in Havana, Cuba. Well, duh, you might be saying to yourself. Everyone knows how much ministers in Massachusetts love their rum, right? Not so fast. Both salaries and rum prices have increased, true, but so has the price of just about everything else (Huff & Geis, 1993). [5] Finally, research shows that as the number of firefighters on the scene increase, the amount of damage caused at the scene increases. Of course, this statement does not mention that the size of the fire impacts the amount of damage and the number of firefighters dispatched to the scene (Frankfort-Nachmias & Leon-Guerrero, 2011). [6] In each of these examples, it is the presence of a third variable that explains the apparent relationship between the two original variables.
In sum, the following criteria must be met for a correlation to be considered causal:
- The two variables must vary together.
- The relationship must be plausible.
- The cause must precede the effect in time.
- The relationship must be nonspurious (not due to a third variable).
Once these criteria are met, a researcher can say they have achieved a nomothetic causal explanation, one that is objectively true. It’s a difficult challenge for researchers to meet. It is extremely uncommon to hear researchers state that they have proven their hypotheses. A statement that bold implies that a relationship has been shown to exist with absolute certainty and that there is no chance that there are conditions under which the hypothesis would not be true. Instead, researchers tend to say that their hypotheses have been supported (or not). This way of discussing findings is more cautious and leaves room for the potential discovery of new evidence or new ways to examine the relationship. Researchers may also discuss a null hypothesis. We covered in Chapter 3 that the null hypothesis is one that predicts no relationship between the variables being studied. If a researcher rejects the null hypothesis, they are stating that the variables in question are somehow related to one another.
Idiographic causal relationships
Remember our question, “Are you trying to generalize… or nah?” If you answered no, you are trying to establish an idiographic causal relationship. I can guess that if you are trying to establish an idiographic causal relationship, you are likely going to use qualitative methods, reason inductively, and engage in exploratory or descriptive research. We can understand these assumptions by walking through them, one by one.
Researchers seeking idiographic causal relationships are not trying to generalize, so they have no need to reduce phenomena to mathematics. In fact, reducing the social world to the language of mathematics can be problematic, as it robs the causal relationship of its meaning and context. Idiographic causal relationships are bound within people’s stories and interpretations. Usually, these are expressed through words. Although most qualitative studies use word data, some can use interpretations of visual or performance art.
But wait, I predicted that an idiographic causal relationship would use descriptive or exploratory research. How can we build causal relationships if we are just describing or exploring a topic? Wouldn’t we need to do explanatory research to build any kind of causal explanation? Explanatory research attempts to establish nomothetic causal relationships—an independent variable is demonstrated to cause changes in a dependent variable. Exploratory and descriptive qualitative research contains some causal relationships, but they are actually descriptions of the causal relationships established by the participants in your study. Instead of saying “x causes y,” your participants will describe their experiences with “x,” in which they will explain the various contributing factors that depend on time, environment, and subjective experience. As we stated before, idiographic causal explanations are messy. Your job as a social science researcher is to accurately describe the patterns in what your participants tell you.
Let’s consider an example. What might you say if I asked you why you decided to become a social worker? For me, I would say that I wanted to be a mental health clinician since I was in high school. I was interested in how people thought. At my second internship in my undergraduate program, I got the advice to become a social worker because the license provided greater authority for insurance reimbursement and flexibility for career change. While that is not a simple explanation, my description provides a deeper understanding of the myriad factors that led me to become a social worker. If we interviewed many social workers about the factors that led them to the field, we might begin to notice patterns. We might find out that many social workers begin their careers based on a variety of factors, such as: personal experience with a disability or social injustice, positive experiences with social workers, or a desire to help others. No one factor is the “most important factor,” like with nomothetic causal relationships. Instead, a complex web of factors, contingent on context, emerge in the dataset when you interpret what people have said.
As you’ll remember from Chapter 6, inductive reasoning is all about finding patterns in data. In a research project using inductive reasoning, a researcher collects data, usually in the form of words, and looks for patterns. Those patterns inform the theories we utilize in social work. In many ways, the idiographic causal relationships you create in qualitative research are like the social theories we reviewed in Chapter 6 (e.g. social exchange theory) and other theories you use in your practice and theory courses. Theories are explanations about how different concepts are associated with each other how that network of relationships works in the real world. While you can think of theories like Systems Theory as Theory (with a capital “T”), inductive causal relationships are like theory with a small “t.” They may apply only to the participants, environment, and moment in time in which you gathered your data. Nevertheless, they contribute important information to the body of knowledge on the topic you studied.
Over time, as more qualitative research is completed and patterns emerge across different studies and locations, more sophisticated theories emerge that explain phenomena across multiple contexts. In this way, qualitative researchers use idiographic causal explanations for theory building or the creation of new theories based on inductive reasoning. Quantitative researchers, on the other hand, use nomothetic causal relationships for theory testing, wherein a hypothesis is created from existing theory (big T or small t) and tested mathematically (i.e., deductive reasoning).
If you plan to study domestic and sexual violence, you will likely encounter the Power and Control Wheel. [6] The wheel is a model of how power and control operate in relationships with physical violence. The wheel was developed based on qualitative focus groups conducted by sexual and domestic violence advocates in Duluth, MN. While advocates likely had some tentative hypotheses about what was important in a relationship with domestic violence, participants in these focus groups provided the information that became the Power and Control Wheel. As qualitative inquiry takes place and researchers learn from their participants, hypotheses become clearer and more specific.
Once a theory is developed from qualitative data, a quantitative researcher can seek to test that theory. For example, a quantitative researcher may hypothesize that men who hold traditional gender roles are more likely to engage in domestic violence. That would make sense based on the Power and Control Wheel model, as the category of “using male privilege” speaks to this relationship. In this way, qualitatively-derived theory can inspire a hypothesis for a quantitative research project.
Unlike nomothetic causal relationships, there are no formal criteria for establishing causality in idiographic causal relationships. In fact, some criteria like temporality and nonspuriousness may be violated. For example, if an adolescent client says, “It’s hard for me to tell whether my depression began before my drinking, but both got worse when I was expelled from my first high school,” they are recognizing that oftentimes it’s not so simple that one thing causes another. Sometimes, there is a reciprocal relationship where one variable (depression) impacts another (alcohol abuse), which then feeds back into the first variable (depression) and also into other variables (school). Other criteria, such as covariation and plausibility, still make sense because the relationships you highlight in your idiographic causal explanation should still be plausibly true and its elements should vary together.
Similarly, idiographic causal explanations differ in terms of hypotheses. If you recall from the last section, hypotheses in nomothetic causal explanations are testable predictions based on previous theory. In idiographic research, a researcher likely has hypotheses, but they are more tentative. Instead of predicting “x will decrease y,” researchers will use previous literature to identify concepts that might be important to participants and how participants might respond during the study. Based on an analysis of the literature, a researcher may formulate a few tentative hypotheses about what they expect to find in their qualitative study. Unlike nomothetic hypotheses, these are likely to change during the research process. As the researcher learns more from their participants, they might introduce new concepts that participants talk about. Because the participants are the experts in idiographic causal relationships, a researcher should be open to emerging topics and shift their research questions and hypotheses accordingly.
Two different baskets
Idiographic and nomothetic causal explanations form the “two baskets” of research design elements pictured in Figure 7.4 below. Later on, they will also determine the sampling approach, measures, and data analysis in your study.
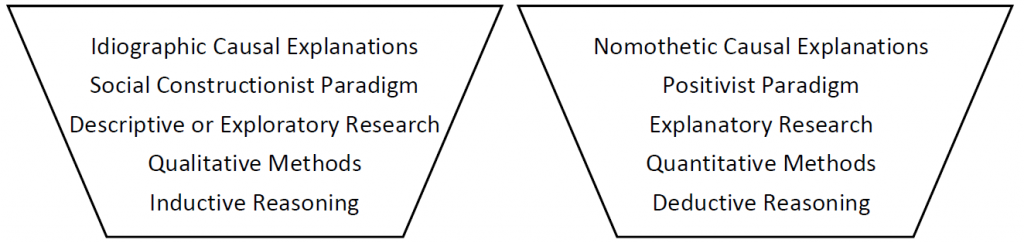
In most cases, mixing components from one basket with the other would not make sense. If you are using quantitative methods with an idiographic question, you wouldn’t get the deep understanding from participants’ own words that you need to answer an idiographic question. For example, knowing that someone scored 20/35 on a numerical index measuring depression symptoms does not tell you what depression means to that person. Similarly, qualitative methods are not often used to deductive reasoning because qualitative methods usually seek to understand a participant’s perspective, rather than test what existing theory says about a concept.
However, these are not hard-and-fast rules. There are plenty of qualitative studies that attempt to test a theory. There are fewer social constructionist studies with quantitative methods, though studies will sometimes include quantitative information about participants. Researchers in the critical paradigm can fit into either bucket, depending on their research question, as they focus on the liberation of people from oppressive internal (subjective) or external (objective) forces.
Later, we will explore how mixed methods researchers can use both buckets simultaneously, but for now, it’s important that you understand the logic that connects the ideas in each separate bucket. Not only is this fundamental to understand how knowledge is created and tested in social work, but it also speaks to the assumptions and foundations that social theories are built on!
Key Takeaways
- Idiographic research focuses on subjectivity, context, and meaning.
- Nomothetic research focuses on objectivity, prediction, and generalization.
- In qualitative studies, the goal is generally to understand the multitude of causes that account for the specific instance the researcher is investigating.
- In quantitative studies, the goal may be to understand the more general causes of some phenomenon rather than the idiosyncrasies of one particular instance.
- To establish a nomothetic causal relationship:
- 1) the relationship must be plausible
- 2) the relationship must be nonspurious
- 3) the cause must precede the effect in time
- 4) the independent variable must cause changes in the dependent variable
- Hypotheses are statements, drawn from theory, which describe a researcher’s expectation about a relationship between two or more variables.
- Qualitative research may create theories that can be tested quantitatively.
- The choice of idiographic or nomothetic causal relationships requires a consideration of methods, paradigm, and reasoning.
- Depending on whether you seek a nomothetic or idiographic causal explanation, you are likely to employ specific research design components.
Glossary
Causality-the idea that one event, behavior, or belief will result in the occurrence of a subsequent event, behavior, or belief
Control variables– the effects of potential “third variables” are mathematically controlled in the data analysis process to highlight the relationship between the independent and dependent variable; used to establish the criteria of nonspuriousness in nomothetic causal relationships
Covariation– the degree to which two variables vary together
Dependent variable– a variable that depends on changes in the independent variable
Generalize– to make claims about a larger population based on an examination of a smaller, representative sample
Hypothesis– a statement describing a researcher’s expectation regarding what they anticipate to find
Idiographic research– attempts to exhaustively explain or describe a phenomenon, based on the subjective understandings of the participants
Independent variable– a variable that causes a change in the dependent variable
Nomothetic research– provides a more general, sweeping explanation that is universally true for all people
Plausibility– in order to make the claim that one event, behavior, or belief causes another, the claim has to make sense
Spurious relationship– an association between two variables appears to be causal but can in fact be explained by some third variable
Statistical significance– the likelihood that the relationships that are observed are caused by something other than chance; the level of confidence that researchers have in a mathematical relationship
Temporality– whatever cause a researcher identifies must happen before the effect
Theory building– the creation of new theories based on inductive reasoning
Theory testing– when a hypothesis is created from existing theory and tested mathematically
Image attributions
Weather TV Forecast by mohamed_hassan CC-0
Beatrice Birra Storytelling at African Art Museum by Anthony Cross public domain
- Uggen, C., & Blackstone, A. (2004). Sexual harassment as a gendered expression of power. American Sociological Review, 69, 64–92. ↵
- In fact, there are empirical data that support this hypothesis. Gallup has conducted research on this very question since the 1960s. For more on their findings, see Carroll, J. (2005). Who supports marijuana legalization? Retrieved from http://www.gallup.com/poll/19561/who-supports-marijuana-legalization.aspx ↵
- Figures 7.2 and 7.3 were copied from Blackstone, A. (2012) Principles of sociological inquiry: Qualitative and quantitative methods. Saylor Foundation. Retrieved from: https://saylordotorg.github.io/text_principles-of-sociological-inquiry-qualitative-and-quantitative-methods/ Shared under CC-BY-NC-SA 3.0 License (https://creativecommons.org/licenses/by-nc-sa/3.0/) ↵
- Babbie, E. (2010). The practice of social research (12th ed.). Belmont, CA: Wadsworth. ↵
- Huff, D. & Geis, I. (1993). How to lie with statistics. New York, NY: W. W. Norton & Co. ↵
- Frankfort-Nachmias, C. & Leon-Guerrero, A. (2011). Social statistics for a diverse society. Washington, DC: Pine Forge Press. ↵