Janet Taylor
Introduction
COVID-19 quickly forced society into a frenzy to stop its spread. Due to its high contagiousness, it is important to know who has potentially been exposed to the virus and who is most likely to develop severe symptoms. Various methods have been developed to collect this information and make it available for researchers and doctors to track COVID-19’s spread. Many medical researchers are looking towards artificial intelligence and electronic contact tracing as the solutions. However, both methods have high potential to violate the privacy of individuals. How much data they should be able to collect is also a contentious issue that is subject to debate. If conducted poorly, more information than necessary may be collected. Information may also end up in the hands of people with ulterior motives. In their responses to the COVID-19 pandemic, governments and large corporations around the world have enacted a series of privacy-eroding measures. They also created invasive technologies that may leave long-lasting consequences on societies long after the pandemic is over.
Connection to Science Technology and Society (STS) – Path Dependency
The threat of technological invasion not being rolled back is explained by path dependency theory. Path dependency theory states that decisions are difficult to change once they have been enacted. As people become more accustomed to a new law or device, it only becomes even harder to change it than before, regardless if the new change would be an improvement over the system currently in place. Once the pandemic is controlled, the ideal change would be to remove the excessive monitoring of public spaces and other social control measures. However, if people have already gotten used to dealing with them, they would consider it an inconvenience to have things reverted to how they were before the pandemic started. Therefore, it is important that privacy invasions never become “normal” to people.
Contact Tracing
Contact tracing is when a device (usually a personal cellphone) tracks a person and who they have come into contact with. If someone in an area becomes infected with the Coronavirus, the device will inform everyone in their vicinity that a person near them has tested positive and will prompt them to go into isolation for fourteen days. There are two main components that make contact tracing function. Somehow, the device must have a way of identifying everyone and must know who to inform in the case of an outbreak. The device must also know its general geographical location.

Data stored for identification purposes can be stored in a decentralized or centralized database. To help picture the difference between the two, consider a situation where two friends meet up to shop together for multiple hours. They each have a contact-tracing app on their phones. The length of the shopping trip gives the friends high risk of transmitting the virus to each other, so each of their phones logs this interaction. Later, one of the friends becomes infected with COVID-19 and registers themselves as such in the app. In a centralized system, the server would know the infection status (and possibly other medical information) of everyone using the app. It would also know the places they have visited and the people they have met. The server makes the final decision on who to notify based on whatever criteria the developers have set. The most important takeaway is that a remote server acts as a middleman and handles almost every part of the transaction. In a decentralized system, the server only knows the unique identifiers for the phones and the infection status of their owners. The app stores the identifiers the user has come into contact with on the phone itself, and routinely checks in with the server to see if any of the local identifiers belong to infected users. The app then notifies the phone’s owner that they may have been exposed.
The debate between centralized and decentralized systems caused a disagreement between some important pillars in STS: Apple, Google, and the National Health Service (NHS), the UK’s governmental health organization. The NHS argued that centralized systems would allow them to determine who should be notified and push out updates easier. The two tech giants advocated for decentralized models, claiming that those models reduced the toll on battery that contact tracing can have and benefitted personal privacy. After severe backlash from both security experts and the public, the NHS did eventually agree to switch to a decentralized model (Kelion).
Centralized systems offer more opportunities for privacy violations than decentralized systems, but they are favored among governments. It is hard or even impossible for civilians to know what is taking place on a remote server after the data is sent, but local apps can be reverse engineered to discover how they work. Ideally, applications should also be designed to store only the information they need. Decentralized systems achieve the same goals as centralized systems – to let people know if they are at risk – and require less data to be sent through a middleman. Decentralized systems are also less vulnerable to hacking or abuse due to the limited amount of data stored on the server. Adoption of either system varies from country to country, however.
Contact tracing applications must have a way of knowing the location of the user in order to inform them if they have been exposed. Dr. Schmidtke, a research fellow at Hanse-Wissenschaftskolleg in Germany, defines an application providing data based on a user’s location as being “location-aware” (Schmidtke). Often, developers will go the path of least resistance when creating applications. They choose to simply send the user’s location, along with whatever other information they are sending to the server at the time. This is more likely to be used in a centralized system, where most transactions are handled by the server anyway. However, this is not even slightly a privacy-centric approach to contact tracing. By giving their detailed location information to a centralized server, users are opening themselves up to being tracked much more than is necessary for contact tracing to be successful. They only have the promises of the service provider (e.g. the government) that that information will not be sold or transferred to other organizations. This assertion is rather unconvincing since the United States government has already been shown to share Coronavirus data with Palantir Technologies, an organization that has helped facilitate deportations and military activity (Piller).
Technology does not develop in a vacuum, and it should be created with potential societal abuses of it in mind. The public cannot rely on “trust” when offering up their information; and it is critical that developers take privacy-centric approaches when creating apps so that this data is not collected in the first place. Contact tracing applications must carefully tread the line between privacy and the health of their users.
Artificial Intelligence
Artificial intelligence, or AI, is when computers are given a set of starting data and a target. They have to fill in the steps to get to the target. Many institutions and researchers have looked to AI for help in tracking and predicting the spread of COVID-19, but thus far, its use has been fairly limited. In order to function, AI must be given data to train on so a model can be developed. This can pose a problem for maintaining privacy of individuals, as that training data must come from somewhere before it is applied to other real-world situations. Care must also be taken to ensure that the training data is not overly biased. AI only knows what it has been fed; so if human biases are given to it, it will produce similarly biased results. AI is also notoriously inflexible. It is not at all equipped to handle events outside of its limited scope of performance, and when a major event like a pandemic occurs, “information overload” becomes a serious issue (Naude). A hot topic like a pandemic makes it very hard for useful data to be found in the chaos of all the discussion.
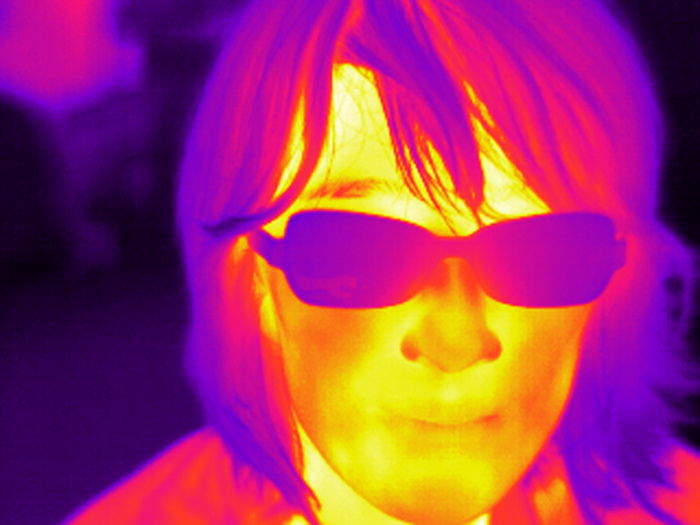
Of course, artificial intelligence is not useless when it comes to the COVID-19 pandemic. Some developers have found promising results with the technology. Researchers from the Massachusetts Institute of Technology (MIT) have created an algorithm that could detect if a person had COVID-19 by their cough. They collected 70,000 audio samples and claim to have a 98.5% success rate, though they currently do not have any authority to develop this product further (Kleinman). AI has been shown to accurately utilize CT scans and X-rays to determine if someone has the virus (Naude). The future of AI seems to be in diagnosing symptoms and the severity of the COVID-19 infection in a person more than it is in combating the semi-unpredictable nature of the spread of the virus itself. It is important to stress that that is not a negative thing, as any help is appreciated when trying to combat the pandemic.
Unfortunately, human recognition using AI brings up severe privacy concerns. Long before the pandemic started, AI was already being used to produce “deepfakes” (realistic-looking fake images or videos of a person) and to recognize human features in photographs. Therefore, it already had quite the head start when it came to recognizing human faces in video footage. AI can easily be used to detect if someone is wearing a mask. Thermal recognition, i.e. using AI to detect if someone has a fever, is used in conjunction with facial recognition worldwide. The goal expressed by those using these recognition methods is to alert nearby people or location staff that someone has violated health guidelines. This can quickly get very invasive and out of hand. In China, those who disobeyed orders would “get a call from the authorities, presumably after being tracked by the facial recognition system.” In China, those who disobeyed orders would “get a call from the authorities, presumably after being tracked by the facial recognition system” (Qtd. in Naude). Companies in the United States and other countries have admitted to using facial recognition to discipline employees who violated social distancing guidelines. This type of automated enforcement of in-person guidelines can pave a dangerous road for future methods of controlling people in public places.
Government’s Management of Data

It cannot be denied that extreme monitoring of citizens can be effective in combating the virus. Some Asian countries, like China, have reported great successes with their intrusive methods of tracking the virus’s spread. Countries are collecting data from any venue they can find, even mobile networks (BBC Editors). Nevertheless, considering these privacy invasions a necessity in the moment can lead to severe harm for our future. Who can say if or when these measures will be rolled back? It is trivial to expand the usage of facial recognition and contact tracing after the pandemic is over. The technology is already installed in cameras and cellphones everywhere. Even the data that is already collected can be used for ulterior motives. Personal information stored in healthcare systems have been used to help with the fight against COVID-19. While this cause is understandable, this same data can be used for more negative causes like the social control and deportation mentioned in previous sections. There are no limits once it is in a company’s or the government’s hands, no matter what cause they give for the data collection.
Despite all the information being collected in the name of effectively countering the virus, some doctors still argue that they are receiving incomplete datasets from their governments. Charles Piller of Science magazine chronicled the complains of doctors and researchers from several institutions. They argued that privacy is not a major concern because the data can be “de-identified.” Though the risk of someone being identified from this data is slim, the same risk is present with any other publicly available dataset if a person is determined enough to track someone. To Rajiv Bhatia, a professor at Stanford University in California, it seems like their state government is not making a genuine effort to provide the data at all. Some researchers in states with looser restrictions on shared information (Like Florida, where residents are provided with information such as age brackets, residential status, and totals by city) still argue that what they are given is not enough (Labs). Epidemiologist Thomas Hladish argued that the more inclusive datasets given by the Floridian government are inadequate for creating unique plans to target the pandemic in local areas (Piller). Ultimately, it is a question of how much individual privacy should be traded in exchange for the health of everyone. While there is no simple, straightforward answer, there is certainly no excuse for disregarding privacy entirely. A line must be drawn somewhere.
Conclusion
Different people have different tolerances for privacy violations. Demographics and prior political beliefs determine how individuals view COVID-19 pandemic prevention measures (Wnuk et al.). STS is clearly expressed in the back-and-forth debates over what technologies should be developed and employed and which should be avoided. Technology can make people feel safe by assisting in things like vaccine development and symptom tracking, but it can also make people feel afraid by creating an excuse for government surveillance and social media witch hunts to occur. We cannot consider only the development of anti-pandemic technologies or only the worries of privacy violations, but must find a way to balance the two. Though the balance can be delicate at times, acceptable trade-offs between safety and privacy can and must be reached.
References
BBC Editors. “Coronavirus: State Surveillance ‘A Price Worth Paying’.” BBC News, 23 Apr. 2020, www.bbc.com/news/technology-52401763.
Kelion, Leo. “NHS Rejects Apple-Google Coronavirus App Plan.” BBC News, 27 Apr. 2020, www.bbc.com/news/technology-52441428.
Kleinman, Zoe. “Algorithm Spots ‘Covid Cough’ Inaudible to Humans.” BBC News, 2 Nov. 2020, www.bbc.com/news/technology-54780460.
Labs, Jennifer, and Sharon Terry. “Privacy in the Coronavirus Era.” Genetic Testing and Molecular Biomarkers, vol. 24, no.9, 2020, pp.535-536, doi:10.1089/gtmb.2020.29055.sjt.
Naudé, Wim. “Artificial Intelligence vs COVID-19: Limitations, Constraints and Pitfalls.” Ai & Society, vol. 35, no. 3, 2020, pp. 761-765, doi:10.1007/s00146-020-00978-0.
Piller, Charles. “Data Secrecy May Cripple U.S. Attempts to Slow Pandemic.” Science, vol. 369, no. 6502, pp. 356-358, science.sciencemag.org/content/369/6502/356.
Schmidtke, H.R.. “Location-Aware Systems Or Location-Based Services: A Survey with Applications to CoViD-19 Contact Tracking.” Journal of Reliable Intelligent Environments, vol. 6, no. 4, 2020, pp. 191-214, doi:10.1007/s40860-020-00111-4.
Wnuk, Anna, et al. “The Acceptance of Covid-19 Tracking Technologies: The Role of Perceived Threat, Lack of Control, and Ideological Beliefs.” PLoS ONE, vol. 15, no. 9, 2020, pp. 1–16, doi:10.1371/journal.pone.0238973.
Images
“Corona Covid-19 App” by viarami is in the Public Domain, CC0
“Self portrait with thermal imager” by Nadya Peek is licensed under CC BY 4.0
“Regulation GDPR Data Protection” by TheDigitalArtist is in the Public Domain, CC0