Personalising Learning
25 AI-Speak: How Adaptive Systems Learn the Learner, part 2
The Process
In recent years, machine learning is increasingly being used in adaptive systems, either as the sole technology or in conjunction with other approaches2. Where used, the principal role of ML is in creating and updating student models, based on a set of features, including results from assessments and new data generated in the process1.
For the outer loop, models are created with the help of training data, by assigning suitable weights –these are features that help recommend effective learning content2. (Also refer to how machine learning works.). These models are used to regularly recommend new learning paths that reflect student progress and changing interests – like new recommendations in Youtube. In ML-based ALS, the number of pathways can run to trillions3.
In the inside loop, machine learning is used to give suitable feedback, spot errors, infer gaps in knowledge and assess mastery of knowledge units: While working on one activity, a student might make errors. ML can be used to predict what errors rise from which knowledge gap. If one step of the solution is correct, ML can be used to predict which knowledge units have been mastered successfully2.
Other techniques used in adaptive systems involve less automation and more explicitly written rules for making inferences2.
They demand a lot of programming time and extra effort to accurately capture all the criteria that go into decision-making. Further, the results cannot often be generalised from one domain to the next, or from one problem to the next.
Tools that use ML use large sets of data on actual student performance and are able, over time, to create the most dynamic learning paths for students1. As in all ML applications, there is training and testing to be done before being put to use in classrooms.
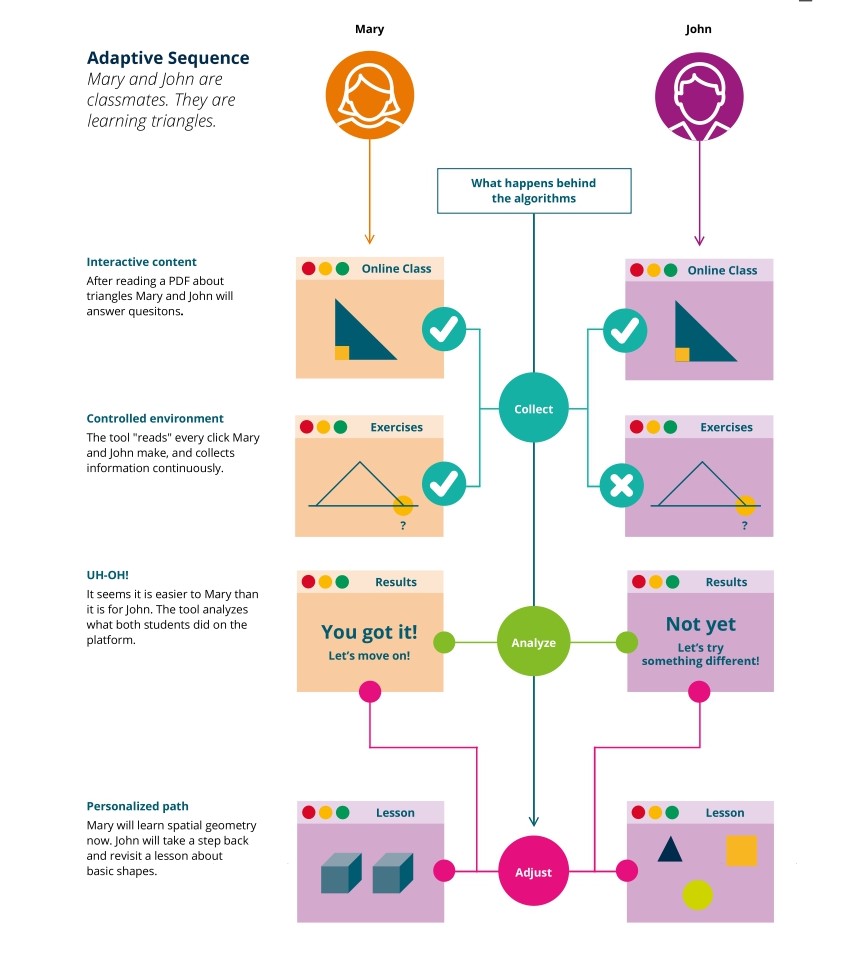
Pedagogical model
In the case of Youtube, we saw there are a lot of value judgements on what makes a good recommendation – such as how many user interests need to be covered in one set of recommendations, how many videos should be similar to already watched ones, how much new content to add for diversity (see How Youtube learns you, part 2). ALS involves similar judgements on what it means to master a KU and how to get to that mastery: the pedagogy and daily experience of the learner4.
In the case of ALS, these judgements and guidance on how a learner is to progress should be based on proven pedagogical theories. These go into the pedagogical model, and, along with the domain and learner models, help the machine to choose an appropriate set of activities.
Some of the questions answered in this model are: should the student next be presented with a concept, an activity or a test? At what level of difficulty? How do they evaluate the learning and provide feedback? Where is more scaffolding necessary5? (Scaffolds are support mechanisms that give guidance on concepts and procedure, the strategy used and how to reflect, plan and monitor learning.) The pedagogical model dictates the breadth and depth of activities – and even whether to continue within the ALS or get help from the teacher3.
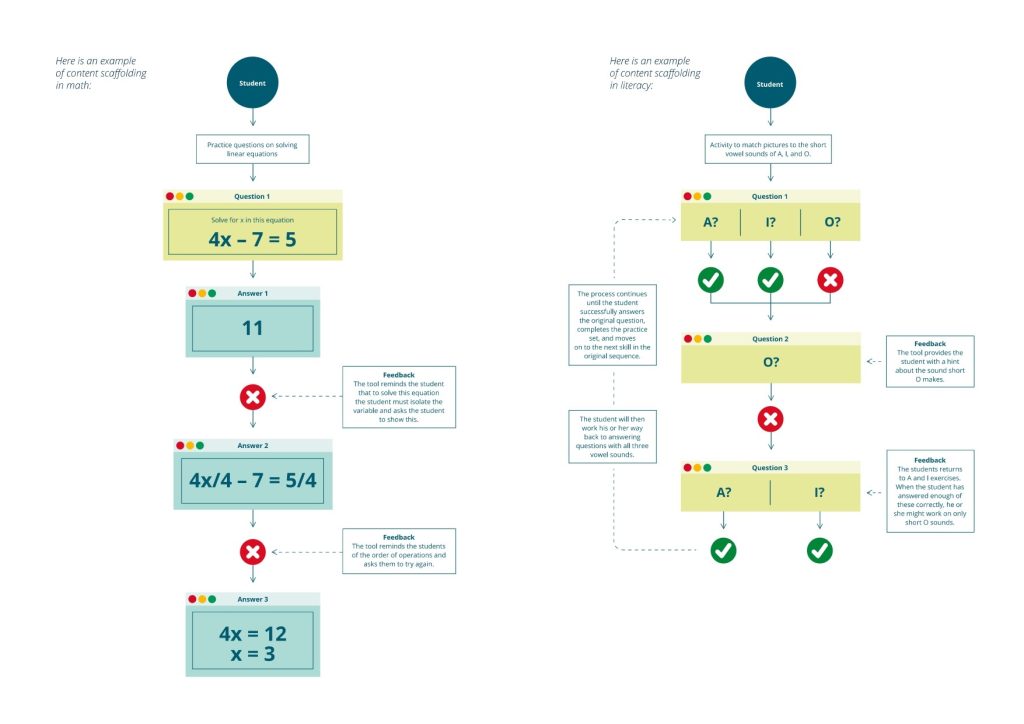
The interface
The recommendations are presented along with other data such as learner progress, performance and goals. The key questions here are:
- How will content be delivered?
- How much content will be recommended in one go?
- What is assigned directly and what is recommended?
- What are the supporting resources?
- Is it possible to provide group activities?
- How much autonomy should be permitted?
- Can students change their preferences?
- Can teachers change the learning pathways?
- What data is shown to the teachers?
- Are the teachers in the loop?
Evaluation
When the ALS is put to use, most systems monitor their own performance against criteria set by the programmer. Like in any AI tool, data might be biased. Inferences drawn by the system can be imprecise. The student’s past data will become less relevant with time6. Therefore, the teacher also has to monitor the system’s performance and provide learner guidance and corrective measures where necessary.
Teachers and peers also have to provide inspiration and reveal alternative resources. research in recommendation systems was shaped by commercial content providers and online retail companies for over a decade. Thus, the focus has been on providing recommendations that produce results that can be promoted. “The surprising delight of an unexpected gem”7 and the allure of roads less travelled, can inspire enduring learning. Unfortunately, these are not the strong points of machine-based personalised learning.
1 EdSurge, Decoding Adaptive, Pearson, London, 2016.
2 Chrysafiadi, K., Virvou, M., Student modeling approaches: A literature review for the last decade, Expert Systems with Applications, Elsevier, 2013.
3 Essa, A., A possible future for next generation adaptive learning systems, Smart Learning Environments, 3, 16, 2016.
4 Groff, J., Personalized Learning: The state of the field and future directions, Center for curriculum redesign, 2017.
5 Alkhatlan, A., Kalita, J.K., Intelligent Tutoring Systems: A Comprehensive Historical Survey with Recent Developments, International Journal of Computer Applications 181(43):1-20, March 2019.
6 du Boulay, B., Poulovasillis, A., Holmes, W., Mavrikis, M., Artificial Intelligence And Big Data Technologies To Close The Achievement Gap, In: Luckin, Rose ed. Enhancing Learning and Teaching with Technology. London: UCL Institute of Education Press, pp. 256–28, 2018.
7 Konstan, J., Terveen, L., Human-centered recommender systems: Origins, advances, challenges, and opportunities, AI Magazine, 42(3), 31-42, 2021.