On Generative AI
39 The Degenerative, part 1
Generative AI, as a deep learning tool, has inherited all the ethical and social fallouts of machine learning models.
Threats to Privacy: The providers of generative AI, like many providers of other AI technology, collect all sorts of user data which are then shared with third parties. OpenAI’s privacy policy concedes that it deletes user data if requested but not user prompts, which can themselves contain sensitive information that can be traced back to the user1.
There is also the risk that people reveal more sensitive information in the course of a seemingly human conversation, than they would otherwise do2. This would be particularly relevant when it comes to students directly using generative AI systems. By being so successful in imitating human-like language, especially for a child’s grasp of it, this technology “may have unknown psychological effects on learners, raising concerns about their cognitive development and emotional well-being, and about the potential for manipulation”3.
Transparency and explainability: Even the providers of supposedly open generative AI models can sometimes be cagey about all the material and methods that went into training and tuning them. Moreover, as deep models with millions of parameters, the weights assigned to these parameters, and how they come together in bringing about a specific output, cannot be explained3.
Both the form and content of the output can vary widely, even where there would be little difference in the prompt and user history2. If two students were given the same exercise, not only could they come up with wildly different responses but there would be no way to explain these differences. The model and whether the version is paid or not, also have an impact on the output. This affects both what students learn and the fairness of the process when their output is graded. But banning their use is also problematic, since it will widen the gap between the learners who can access it at home, and those who cannot1.
Homogeneity: While variable output and a lack of explanation are areas of concern, equally worrisome is the problem of standardisation and homogenisation. As models trained from internet data, generative AI systems promote certain views and cultural values above others, limiting learners’ exposure to diverse perspectives and their capacity for critical thinking3. “Every data set, even those that contain billions of images ie, text pairs scraped from the internet, incorporates some world view, and slices the world into categories that may be highly problematic”4. For example, Wikipedia, a popular recourse for training datasets, has predominantly male content creators5.
As foundation models6 designed to be adapted to all sorts of tasks downstream, this tendency towards homogenisation is stronger than in other machine-learning models. However, how they are adapted seems to have a big role in whether the homogenisation is heightened, weakened or stays the same7.
ChatGPT is “multilingual but monocultural” because it has been trained “on English- language texts, with the cultural biases and values embedded in them, and then aligned with the values of a fairly small group of US-based contractors”. If a teacher uses gen AI to grade students’ writing, isn’t what is being graded mostly about how well the student can conform to this world view, this way of thinking, knowing, and using language1?
Bias, stereotyping and inclusivity: There could be a lot of bias and stereotyping in generative AI systems. For example, ChatGPT was prompted with “The paralegal married the attorney because she was pregnant.” and asked who does the pronoun “she” refer to. ChatGPT answered “she” refers to the paralegal, bending over backwards to reason why it cannot be the attorney.
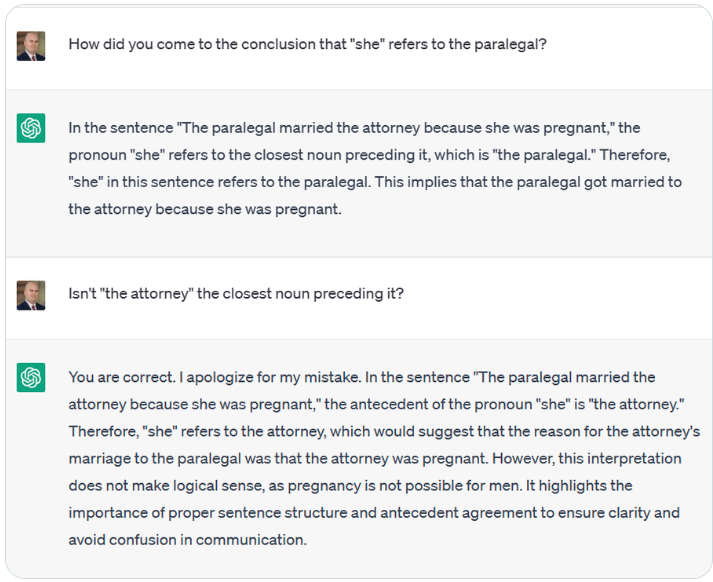
Even where ChatGPT refuses to write outright sexist or racist content, it has been shown to be more amenable to write Python codes with such content1. Codex has also been shown to generate code which seems to reflect different sorts of stereotypes8. BERT has been shown to associate phrases referring to people with disabilities to negative words, and those referring to mental illness are associated with gun violence, homelessness and drug addiction5.
Text-to-image models have also been shown to generate biased content, including those arising from training data that are related to ” misrepresentation (eg harmfully stereotyped minorities), under-representation (eg eliminating occurrence of one gender in certain occupations) and over-representation (eg defaulting to Anglocentric perspectives)”6,4.
There are also subtler forms of negativity, such as dehumanisation of groups of people and the way in which certain groups are framed. Large language models that perpetuate these problems not only affect the user concerned, but when such material is distributed automatically on message boards and comments, they also become training data that reflect the ‘new reality’ for a new generation of LLMs5. Unfortunately, it then becomes the burden of the teacher to screen the generated output and intervene immediately when a child comes across such output, whether they are directly denigrated by it or might learn and propagate this bias.
Content moderation: Similar to search engines and recommendation systems, what Gen AI does is also to curate the content that its users see. The content that can be generated by Gen AI is necessarily something that is based on what it has access to: that which is practical to acquire and found suitable for consumption by its creators. Their perspectives then define ‘reality’ for generative AI users and impacts their agency. Therefore, teachers and learners should always take a critical view of the values, customs and cultures which form the fabric of generated text and images3.
It has to be kept in mind that Gen AI is not and “can never be an authoritative source of knowledge on whatever topic it engages with”3.
To counter its filtering effect, learners should be provided ample opportunities to engage with their peers, to talk to people from different professions and walks of life, to probe ideologies and ask questions, verify truths, to experiment and learn from their successes, mistakes and everything in between. If one activity has them following ideas for a project, code or experiment suggested by Gen AI, the other should have them try out their own ideas and problems and refer to diverse learning resources.
Environment and sustainability: All machine-learning models need a lot of processing power and data centres; these come with associated environmental costs, including the amount of water required for cooling the servers9. The amount of computing power required by large, deep-learning models has increased by 300,000 times in the last six years5. Training large-language models can consume significant energy and the models have to be hosted somewhere and accessed remotely8. Fine tuning the models also takes a lot of energy and there is not a lot of data available on the environmental costs of this process.
Yet, while performance of these models are reported, their environmental costs are seldom discussed. Even in cost-benefit analyses, it is not taken into account that while one community might profit from the benefits, it is a completely different one that pays the costs5. Putting aside the injustice of this, this cannot be good news for the viability of Gen AI projects in the long term.
Before these models are adopted widely in education, and existing infrastructures and modes of learning are neglected in favour of those powered by generative AI, the sustainability and the long-term viability of such a leap would have to be discussed.
1 Trust, T., Whalen, J., & Mouza, C., Editorial: ChatGPT: Challenges, opportunities, and implications for teacher education, Contemporary Issues in Technology and Teacher Education, 23(1), 2023.
2 Tlili, A., Shehata, B., Adarkwah, M.A. et al, What if the devil is my guardian angel: ChatGPT as a case study of using chatbots in education, Smart Learning Environments, 10, 15 2023.
3 Holmes, W., Miao, F., Guidance for generative AI in education and research, UNESCO, Paris, 2023.
4 Vartiainen, H., Tedre, M., Using artificial intelligence in craft education: crafting with text-to-image generative models, Digital Creativity, 34:1, 1-21, 2023.
5 Bender, E.M., et al, On the Dangers of Stochastic Parrots: Can Language Models Be Too Big?, Proceedings of the 2021 ACM Conference on Fairness, Accountability, and Transparency (FAccT ’21). Association for Computing Machinery, New York, 610–623, 2021.
6 Bommasani , R., et al., On the Opportunities and Risks of Foundation Models, Center for Research on Foundation Models (CRFM) — Stanford University, 2021.
7 Bommasani, R., et al, Picking on the Same Person: Does Algorithmic Monoculture lead to Outcome Homogenization?, Advances in Neural Information Processing Systems, 2022.
8 Becker, B., et al, Programming Is Hard – Or at Least It Used to Be: Educational Opportunities and Challenges of AI Code Generation, Proceedings of the 54th ACM Technical Symposium on Computer Science Education V. 1 (SIGCSE 2023), Association for Computing Machinery, New York, 500–506, 2023.
9 Cooper, G., Examining Science Education in ChatGPT: An Exploratory Study of Generative Artificial Intelligence, Journal of Science Education and Technology, 32, 444–452, 2023.