Why Learn About AI
5 Why not just do AI – Part 1
The second extreme position when it comes to AI is the indiscriminate use or misuse of the technology. Artificial intelligence works differently from human intelligence. Whether due to the nature of the situation, its design or the data, AI systems can work differently from what is expected.
For example, an application developed using a set of data for one purpose will not work as well on other data for another purpose. It pays to know the limitations of artificial intelligence and correct for it; it is good to not just do AI but learn about its advantages and limitations.
Perpetuation of Stereotypes
Google Translate learns how to translate from the internet. Its ‘data miners’ scout the public web for data from which to learn. Along with language, AI learns that the number of male mechanics are more than that of female mechanics, and that the number of female nurses eclipses that of male nurses. It cannot differentiate between what is ‘true’ and what is a result of stereotyping and other prejudices. Thus, Google Translate ends up propagating what it learns, cementing stereotypes further1:
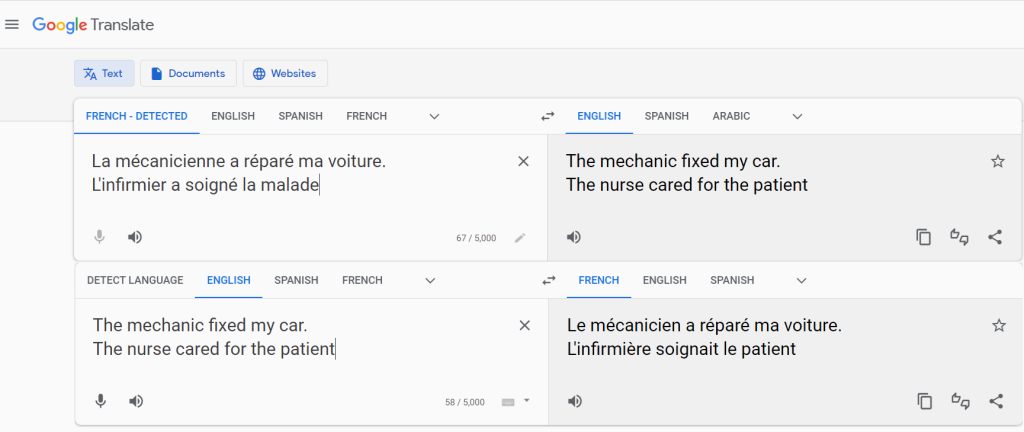
Problems creep up in AI whenever an individual case differs from the majority case (whether this represents faithfully the majority in the real world or just the majority as represented by the internet). In classrooms, the teacher has to compensate for the system’s lapses and, where necessary, direct student’s attention to the alternative text.
Explore
Can you hunt for a stereotype in Google Translate? Play with translating to and from different languages. By clicking on the two arrows between the boxes, you can invert what is being translated (This is what we did for the example shown above).
Languages such as Turkish have the same word for ‘he’ and ‘she’. Many stereotypes come to light when translating from to Turkish and back. Note that many languages have a male bias – an unknown person is assumed to be male. This is not the bias of the application. What is shocking in our example above is that the male nurse is changed into female.
Multiple Accuracy measures
AI systems make predictions on what a student should study next, whether he or she has understood a topic, what group split-up is good for a class or when a student is at risk of dropping out. Often, these predictions have a percentage tagged to them. This number tells us how good the system estimates its predictions to be.
By its nature, predictions can be erroneous. In many applications, it is acceptable to have this error. In some cases, it is not. Moreover, the way this error is calculated is not fixed. There are different measures, and the programmer chooses what he or she thinks is the most relevant. Often, accuracy changes according to the input itself.
Since in a classroom, these systems make predictions on children, it is for the teacher to judge what is acceptable and to act where a decision taken by AI is not appropriate. To do this, a little background on AI techniques and the common errors associated with them will go a long way.
1 Barocas, S., Hardt, M., Narayanan, A., Fairness and machine learning Limitations and Opportunities, MIT Press, 2023.