Managing Learning
19 Issues with Data: Bias and Fairness
Bias is prejudice towards or against an identity, whether good or bad, intentional or unintentional1. Fairness is the counter to this bias and more: when everyone is treated fairly, regardless of their identity and situation. Clear processes have to be set and followed to make sure everyone is treated equitably and has equal access to opportunity1.
Human-based systems often comprise a lot of bias and discrimination. Every person has their own unique set of opinions and prejudices. They too are black boxes whose decisions, such as how they score answer sheets, can be difficult to understand. But we have developed strategies and established structures to watch out for and question such practices.
Automated systems are sometimes touted as the panacea to human subjectivity: Algorithms are based on numbers, so how can they have biases? Algorithms based on flawed data, among other things, can not only pick up and learn existing biases pertaining to gender, race, culture or disability – they can amplify existing biases1,2,3. And even if they are not locked behind proprietary walls, they can’t be called up to explain their actions due to the inherent lack of explainability in some systems such as those based on Deep Neural Networks, .
Examples of bias entering AIED systems
- When programmers code rule-based systems, they can put their personal biases and stereotypes into the system1.
- A data based algorithm can conclude not to propose a STEM-based career path for girls because female students feature less in the STEM graduate dataset. Is the lesser number of female mathematicians due to existing stereotypes and societal norms or is it due some inherent property of being female? Algorithms have no way to distinguish between the two situations. Since existing data reflects existing stereotypes, the algorithms that train on them replicate existing inequalities and social dynamics4. Further, if such recommendations are implemented, more girls will opt for non-STEM subjects and the new data will reflect this – a case of self-fulfilling prophecy3.
- Students from a culture that is under-represented in the training dataset might have different behaviour patterns and different ways of showing motivation. How would a learning analytics calculate metrics for them? If the data is not representative of all categories of students, systems trained on this data might penalise the minority whose behavioural tendencies are not what the program was optimised to reward. If we’re not careful, learning algorithms will generalise, based on the majority culture, leading to a high error rate for minority groups4,5. Such decisions might discourage those who could bring diversity, creativity and unique talents and those who have different experiences, interests and motivations2.
- A British student judged by a US essay correction software would be penalised for their spelling mistakes. Local language, changes in spelling and accent, local geography and culture would always be tricky for systems that are designed and trained for another country and another context.
- Some teachers penalise phrases common to a class or region, either consciously or because of biased social associations. If an essay-grading software trains on essays graded by these teachers, it will replicate the same bias.
- Machine learning systems need a target variable and proxies for which to optimise. Let us say high-school test scores were taken as the proxy for academic excellence. The system will now train exclusively to boost patterns that are consistent with students who do well under the stress and narrowed contexts of exam halls. Such systems will boost test scores, and not knowledge, when recommending resources and practice exercises to students. While this might also be true in many of today’s classrooms, the traditional approach at least makes it possible to express multiple goals4.
- Adaptive learning systems suggest resources to students that will remedy a lack of skill or knowledge. If these resources need to be bought or require home internet connection, then it is not fair to those students who don’t have the means to follow the recommendations: “When an algorithm suggests hints, next steps, or resources to a student, we have to check whether the help-giving is unfair because one group systematically does not get useful help which is discriminatory“2.
- The concept of personalising education according to a student’s current knowledge level and tastes might in itself constitute a bias1. Aren’t we also stopping this student from exploring new interests and alternatives? Wouldn’t this make him or her one-dimensional and reduce overall skills,knowledge and access to opportunities?
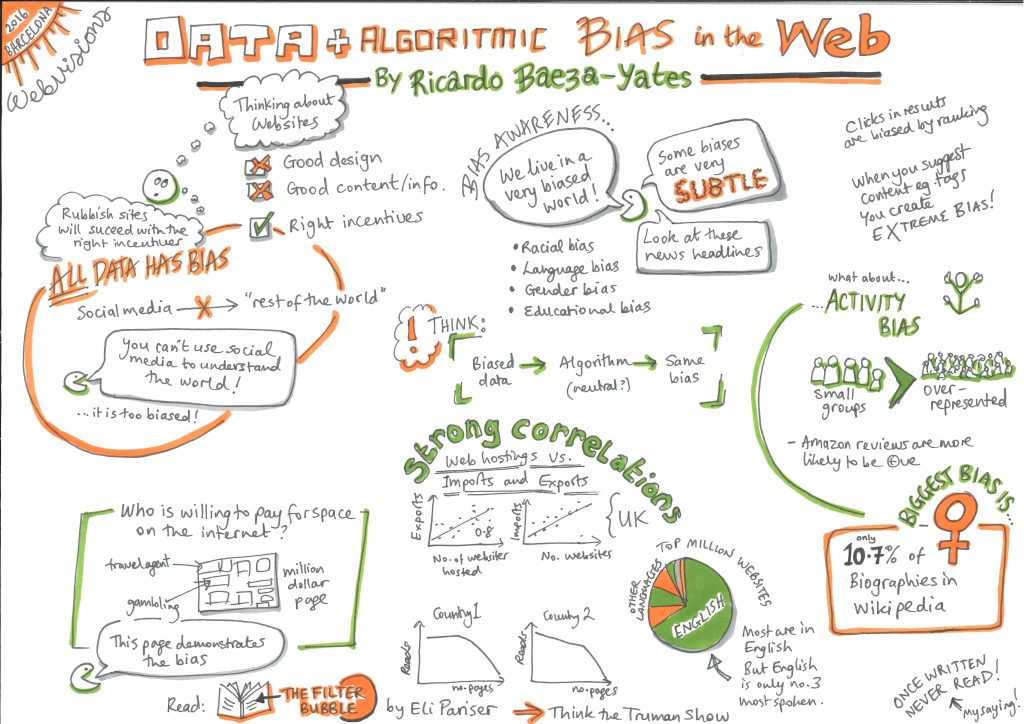
What can the teacher do to reduce the effects of AIED biases?
Researchers are constantly proposing and analysing different ways to reduce bias. But not all methods are easy to implement – fairness goes deeper than mitigating bias.
For example, if existing data is full of stereotypes – “do we have an obligation to question the data and to design our systems to conform to some notion of equitable behaviour, regardless of whether or not that’s supported by the data currently available to us?“4. Methods are always in tension and opposition with each other and some interventions to reduce one kind of bias can introduce another bias!
So, what can the teacher do?
- Question the seller – before subscribing to an AIED system, ask what type of datasets were used to train it, where, by and for whom was it conceived and designed, and how was it evaluated.
- Don’t swallow the metrics when you invest in an AIED system. An overall accuracy of, say, five percent, might hide the fact that a model performs badly for a minority group4.
- Look at the documentation – what measures, if any, have been taken to detect and counter bias and enforce fairness1?
- Find out about the developers – are they solely computer science experts or were educational researchers and teachers involved? Is the system based solely on machine learning or were learning theory and practices integrated2?
- Give preference to transparent and open learner models which allow you to override decisions2, many AIED models have flexible designs whereby the teacher and student can monitor the system, ask for explanations or ignore the machine’s decisions.
- Examine the product’s accessibility. Can everyone access it equally, regardless of ability1?
- Watch out for the effects of using a technology, both long-term and short-term, on students, and be ready to offer assistance when necessary.
Despite the problems of AI-based technology, we can be optimistic about the future of AIED:
- With increased awareness of these topics, methods to detect and correct bias are being researched and trialled;
- Rule-based and data-based systems can uncover hidden biases in existing educational practices. Exposed thus, these biases can then be dealt with;
- With the potential for customisation in AI systems, many aspects of education could be tailored. Resources could become more responsive to students’ knowledge and experience. Perhaps they could integrate local communities and cultural assets, and meet specific local needs2.
1 Ethical guidelines on the use of artificial intelligence and data in teaching and learning for educators, European Commission, October 2022.
2 U.S. Department of Education, Office of Educational Technology, Artificial Intelligence and Future of Teaching and Learning: Insights and Recommendations, Washington, DC, 2023.
3 Kelleher, J.D, Tierney, B, Data Science, MIT Press, London, 2018.
4 Barocas, S., Hardt, M., Narayanan, A., Fairness and machine learning Limitations and Opportunities, MIT Press, 2023.
5 Milano, S., Taddeo, M., Floridi, L., Recommender systems and their ethical challenges, AI & Soc 35, 957–967, 2020.