Additional Content
Cookies and Fingerprinting
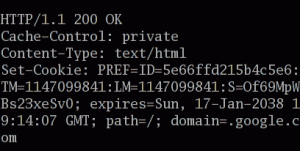
Cookies are small files in your computer that tell the web browser that you are, say, user number #745673 on this website and that you like this and that. These cookies were conceived so that every time we visit the same site, we don’t have to specify preferences such as language and location, lose items in the shopping cart or fill out forms from scratch. In the early stages of this technology, we had full control over what data the cookies could collect1,2.
Afterwards, companies realised they could use cookie data to understand what we like to click on or buy. Thus, ads could be served that did not depend on the content of the current page, but our own personal tastes (behavioural targeting)1. Later, companies began to set their cookies on other company websites to track every user even more closely. These third-part cookies paid the host for this privilege. This is when ads started following us across websites.
Moreover, by using things such as email IDs or credit card numbers, these companies could link the different identification numbers to a single user to have better information on their behaviour. This is called cookie synching. The user of course has no way of knowing what data is being put together to build their behavioural profile.
To add to this, machine learning algorithms started to be put to use to crunch user data and assign them labels like man, woman, black, European or even “prone to depression”1. These labels have nothing to do with our identities, but with what kind of prior user behaviour most resembles that of our own. These labels are sold to companies that sell products, houses and job opportunities. Thus, users with some labels are shown one ad and someone with a different online behaviour a completely different ad in the same web page. This in turn can determine what type of jobs we apply for and in which neighbourhood we buy a house and thus, which schools our children attend3.
Nowadays, cookie technology is embedded into most internet browsers. A 2016 study found that most third parties do cookie syncing. “45 of the top 50, 85 of the top 100, 157 of the top 200, and 460 of the top 1,000” third parties synch cookies from different sources to put together information on users4. It has been shown that Google, for example, can track a user across 80% of websites5 raising threats to privacy and autonomy, and bolstering surveillance and monitoring6.
When these results were published, they raised public outrage. Many cookie-blocking browser plugins became popular, such as DoNotTrackMe. Internet browsers started having controls to block or delete cookies2. Companies such as Apple and Google even stopped or pledged to ban third-party cookies1. Online targeting moved from cookies to more persistent tracking techniques.
For example, cookie-like files could be stored with Adobe’s Flash player; these remain after other cookies have been deleted. These can in turn be blocked by installing apps such as FlashBlock2. Tracking technology is equipped with more persistent tools, such as various types of fingerprinting which are not detected by most blocking tools4.
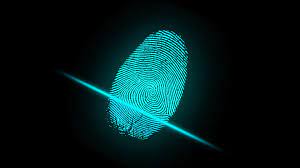
The idea is that our devices and services, such as computers, phones and device speakers, process data and give output slightly differently from other users’ devices. They can serve as our unique fingerprints, especially when the different techniques are put together to create our online identity4. The IP address of our devices, ethernet or Wifi addresses (WebRTC-based finger-printing), how our hardware and software play audio files (AudioContext fingerprinting) and even information on the battery, can all be used as our long- and short-term identifiers that keep online tracking alive7,4.
1 Kant, T., Identity, Advertising, and Algorithmic Targeting: Or How (Not) to Target Your “Ideal User”, MIT Case Studies in Social and Ethical Responsibilities of Computing, 2021.
2 Schneier, B., Data and Goliath : the Hidden Battles to Collect Your Data and Control Your World, W.W. Norton & Company, New York, 2015.
3 Barocas, S., Hardt, M., Narayanan, A., Fairness and machine learning Limitations and Opportunities, 2022.
4 Englehardt, S., Narayanan, A., Online Tracking: A 1-million-site Measurement and Analysis, Extended version of paper, ACM CCS, 2016.
5 Libert, T., Exposing the Invisible Web: An Analysis of Third-Party HTTP Requests on 1 Million Websites, International Journal of Communication, v. 9, p. 18, Oct. 2015.
6 Tavani, H., Zimmer, M., Search Engines and Ethics, The Stanford Encyclopedia of Philosophy, Fall 2020 Edition, Edward N. Zalta (ed.).
7 Olejnik, L., Acar, G., Castelluccia, C., Diaz, C., The leaking battery, Cryptology ePrint Archive, Report 2015/616, 2015.