Clodoaldo Polo Barrera1, María Martínez-Rojas1 and Juan Carlos Rubio-Romero1
1 Universidad de Málaga.
Keywords: Occupational accidents, Health and Safety, Information Systems, Construction Sector, Manufacturing Sector, KNIME platform.
1. Introduction
Occupational accidents are a problem that affects all work sectors. All of them involve a high human and economic cost both for companies and society, although not all of them are of the same severity [1]. In this work, we focus on the application of data mining techniques and data analysis to compare accidents in construction and manu- facturing sectors in order to learn more about previous workplace accidents. These tech- niques allow to contemplate a large number of possible variables related to each acci- dent and allow to find relational patterns between them [2].
2. Methodology
2.1. Database and filtering process
The database contains a high number of variables (58) which characterize the occur- rence of each of the occupational accidents that are collected. The database has been provided by the Ministry of Labor and Social Economy and it contains the accidents that occurred in Spain between 2009-2018. Each year occurs around half a million work accidents, so that in the data set there are about 6 million cases, each with its 58 asso- ciated characteristics. The data set has a size that implies a considerable computational demand for its analysis. To do this, KNIME [3] data mining platform will be used to filter and analyze data.
After the filtering process, the database is reduced from almost 6 million (5,920,749) to a total of 1,744,252 cases belonging to the mentioned sectors, which again filtered by the same type of node to separate construction and industry, obtaining 704,681 cases and 1,039,571 cases, respectively.
2.2. Variables
A set of variables that are of interest to the research community has been selected [4]. The variables are age, temporality, Physical activities, and Type of contact. By analyz- ing these variables, the aim is to respond questions like the 5 W (who, when, what, how, where) to generate knowledge.
3. Multivariable Analysis with decision tree technique
The following analysis will evaluate the variables contained in the data set to deter- mine which ones are the most relevant to predict the accident severity. To do this, de- cision tree technique which is a supervised data mining method that can serve as an effective tool for multivariate data analysis is used [5].
The technique creates a top-down branching structure, consisting of a root node that is split into several branches. This technique provides simplicity and ease of interpre- tation of the results, allowing them to be evaluated from the beginning to the end of the tree visually node by node. Furthermore, decision trees are useful for our evaluated dataset which contains both for quantitative and qualitative variables. Different tech- niques of decision trees modeling have been tested. The one with the best success rate have been the Gain Ratio technique with no pruning for both sectors. With this tech- nique, a result with 88% of accuracy has been obtained.
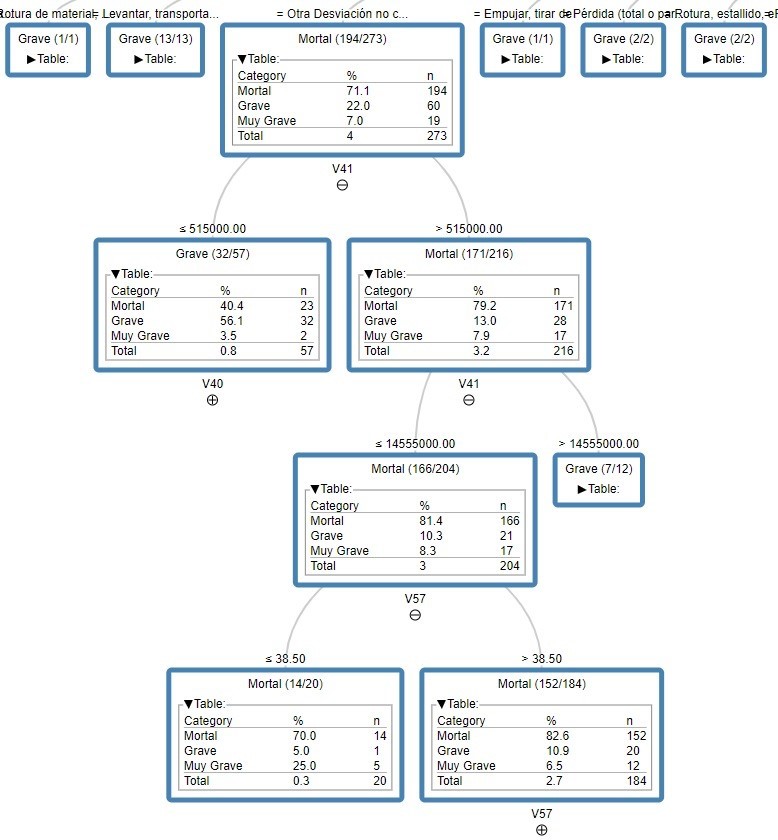
4. Conclusions
Overall, the results obtained for both sectors are similar, although interesting differ- ences have also been found. Regarding to the age, greater differences have been ob- served among workers injured according to their age in evaluations relating to the con- struction sector, while in industry this variable has not been so influential. Regarding the temporality, both sectors have presented fairly similar results, with the greatest dif- ference being found in the results obtained for night work, where the accident rate in construction far exceeds the industry rate.
In relation to the most frequent and relevant causes of accidents for both sectors evaluated through multivariate analysis, it has been observed that human error is in most cases the main cause of the accident. Work activities that may seem more trivial tend to cause the highest number of accidents in both sectors.
The results obtained through the decision trees are also striking, where the ramifica- tions and results of greater success in the prediction for the construction sector have been obtained for deviations not collected by the Delt@ system, a result that is not given for the industry sector.
References
- INSST, “Informe Anual de Accidentes de Trabajo en España,” pp. 1–38, 2019.
- M. Martínez-Rojas, J. A. Torrecilla-García, and J. C. Rubio-Romero, “Prediction Model of Construction Accidents During the Execution of Structures Using Decision Tree Technique,” in Occupational and Environmental Safety and Health II, P. M. Arezes, J. S. Baptista, M. P. Barroso, P. Carneiro, P. Cordeiro, N. Costa, R. B. Melo, A. S. Miguel, and
- Perestrelo, Eds. Cham: Springer International Publishing, 2020, pp. 133–140.
- “Build End to End Data Science,” 2020. Accessed: Feb. 25, 2021. [Online]. Available: www.knime.com.
- Martínez-Rojas, M., Soto-Hidalgo, J. M., Martínez-Aires, M. D., & Rubio-Romero, J. C. (2021). An analysis of occupational accidents involving national and international construction workers in Spain using association rule technique. International journal of occupational safety and ergonomics, 1-37.
- S. Sarkar, A. Patel, S. Madaan, and J. Maiti, “Prediction of Occupational Accidents Using Decision Tree Approach,” 2016.