Ana Maria Peco Chacón1, Isaac Segovia Ramírez1
, and Fausto Pedro García Márquez1
1 Ingenium Research Group, Universidad de Castilla la Mancha, Avda. Campus Universitario S/N, 13071 Ciudad Real (Spain)
AnaMaria.Peco@uclm.es, Isaac.Segovia@uclm.es, FaustoPedro.Garcia@uclm.es
Keywords: Alarm, Machine Learning, Classification Algorithm, Ensemble Tree, Boosted, Bagged, RUSBoosted, Wind Turbine.
1. Introduction
A proactive maintenance management plan is essential to guarantee the availability of wind farms. In recent years, the application of artificial intelligence for fault detection and diagnosis has grown. The volume and variety of data generated by monitoring systems involve advanced analytics based on machine learning algorithms. The main novelty proposed in this work is the use of the ensemble trees algorithm for false alarm classification and detection in wind turbines. A real case study is analyzed to validate the approach.
2. Objectives
The methodology developed for this work is shown in Fig. 1.
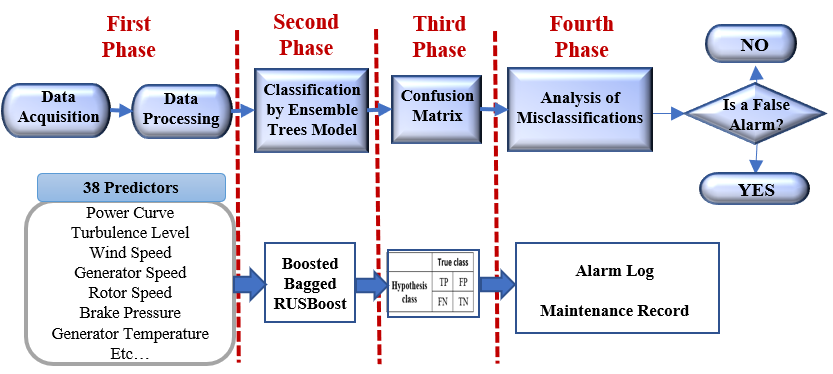
3. Results
This research works with SCADA data from the European project OPTIMUS. For this case study, 38 signals were used as predictors and measured for two months every 10 minutes. The response variable is the alarm log, and two class labels are considered: alarm activation or no alarm activation. Table 1 shows the different methods studied.
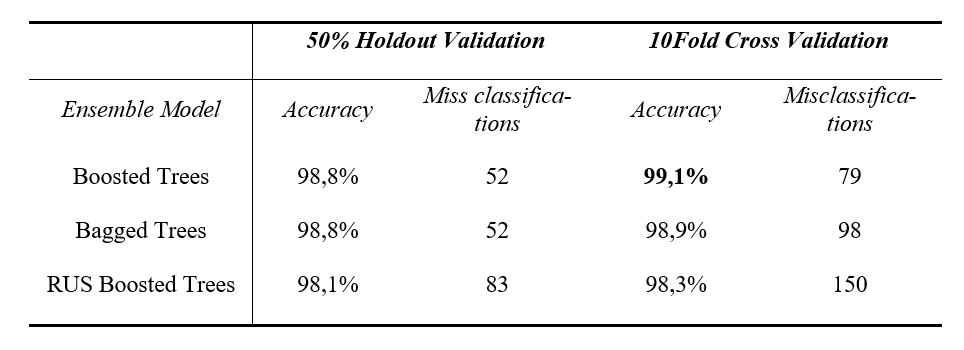
There are 55 misclassifications, defined as False Negative.
- 6 maintenance activities were performed with 4 undetected cases.
- One alarm related to the level of turbulence has been activated 14 times and the method has been unable to detect it.
- At 13 different points, the alarm has only been activated for 11 sec., and it is considered a false alarm produced by the SCADA system because the prediction model is unable to detect it.
- In the rest of the misclassifications, there is a desynchronization between the start or the end of the alarm with respect to the periods predicted by the model. The alarm period is not properly detected by the model in these misclassifications.
The classification model produces false alarms in the prediction, defined as FP points. In this case, there are 24 points and the alarms occurred in these points just before or after the alarm prediction.
4. Conclusions
False alarm identification is a critical process in wind turbine maintenance management. The novelty presented in this paper is the use of the ensemble methods for alarm prediction and classification, as well as the detection of false alarms produced by the system of supervisory control and data acquisition. The ensemble boosted trees model with 10-fold CV presents an accuracy of 99,1%. This method of prediction can reduce the false alarms described as misclassifications by 23,6%. The sensitivity and the specificity are 86,7% and 99,7%, respectively.
Acknowledgements. The work reported herewith has been financially by the Dirección General de Universidades, Investigación e Innovación of Castilla-La Mancha, under Research Grant ProSeaWind project (Ref.: SBPLY/19/180501/000102)